Abstracts for the
NFDI4Ing Conference 2023
Innovation in Research Data Management: Bridging the gaps between disciplines and opening new perspectives for research in engineering science
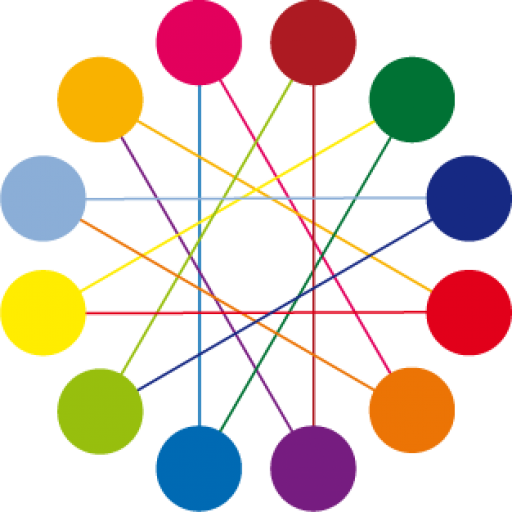
If not, sign up now for the conference 2023!
Abstracts for the NFDI4Ing Conference 2023
To read an abstract, please click its title. You can also collapse the abstract again this way. The ID is solely for purposes of the review process.
Abstracts are sorted by ID.
Jarves: The Digital Data Steward for Engineering Science Research - Tool demonstration
Rising amounts of data and the corresponding management and demands of funding institutions lead to an increasing significance of research data management (RDM), which introduces researchers to new competencies. Researchers must therefore know what specifications they have to meet with their RDM. There is also a demand for information on which tools are available to support them, and where they can receive guidance or training materials on RDM. However, a survey conducted amongst 168 researchers showed that more than half of the interviewees do not know the FAIR principles. Over 60% do not use a data management plan for their research. To foster RDM in engineering, researchers must be informed about the possibilities and practices of successful research data management.
However, researchers are often missing rules, guidance, or related knowledge for their RDM while facing additional effort because of missing automation and repetitive work. In the aforementioned survey, only 22% of the interviewees claimed to have access to RDM specifications, while 39% answered to not have any support at all. The latter statement shows a discrepancy between the interviewees’ perceptions and the existing solutions. For instance, several universities offer RDM support to help researchers get started on RDM. Also, there are training materials either by universities or initiatives such as the NFDI, respectively, included consortia. Conducted interviews showed that solutions and tools offered, as well as the proper usage of these, are often not known by researchers. Numerous researchers wished for more automation in research data management, as RDM itself is often perceived as an additional effort rather than as a benefit. The gap between RDM theory and research practice in the engineering community must be addressed in a way that combines guidance and applicability with existing solutions.
The high initial effort to define or research existing guidelines in RDM for researchers has to be considered along with the missing RDM knowledge amongst researchers. A new solution must be set to provide a benefit in RDM rather than more administrative work. As there are existing solutions, the goal should be to connect them rather than design a new one. To address these challenges, the status quo of RDM in engineering was surveyed, the needs of researchers were scouted via focus group interviews, and, RDM-process-related workshops were conducted. First, a survey on RDM in general and knowledge about it was performed among 168 researchers in the engineering sciences. The goal was to understand the spread and usage of RDM amongst engineering researchers. As a second step, focus group interviews were performed to identify subconsciously or consciously performed RDM tasks amongst the interviewees from engineering sciences.
For example, many manage their code via Git, which already is RDM but was often not considered as such by the interviewees. Thirdly, typical workflows and processes were collected in a workshop format. The workshops were designed to be as open as possible to not set false artificial boundaries to the researchers’ everyday work when designing an RDM process for them.
Lastly, a suitable solution was conceptualised, possible technical solutions were scouted, and a demonstrator was implemented. As a result, a framework, the “Joint Assistant for Research in Versatile Engineering Sciences”, or in short Jarves, was created that aims to address these challenges by providing a structured RDM process with recommendations regarding tools and training materials and partial automation. It is designed as a digital data steward, specifically for research data management in engineering. Jarves provides a guideline for RDM that can be tailored to the specific RDM rules of the researchers’ university, institute, or funding institution. The so structured process guides researchers through their everyday research data management, supporting them not only with steps and tasks to perform but also practical solutions and training materials for RDM issues, readily accessible at the point of need.
Jarves’ integrated decision support considers multiple factors for the hints it provides. For example, not only the institution’s RDM guideline influences the archiving solution. The specific disciplines involved, the amount of data collected, and the presence of personal data have to be taken into account. From the creation of the research data management plan to the archiving of data at the end of the project, Jarves helps researchers manage research data at every stage so that they can focus on their research tasks. Existing online tools in research data management, such as RDMO (Research Data Management Organizer) are integrated into Jarves via API access. This integration allows researchers to switch between different data management tools seamlessly while keeping them synchronized.
The proposed framework shall be demonstrated via the web tool Jarves to inform the engineering community on the one hand and enable the authors to collect feedback to further enhance the framework and its implementation in its development period. Therefore, the demonstration contains a brief overlook of the goal and purpose of Jarves, followed by technical aspects such as architecture and implementation approaches. The latter is meant to give other developers of tools an overview of how to make their tool ready to connect to Jarves, as well as how to connect to Jarves themselves. After that, the current status of the interface implementation is presented along with certain functionalities, including the steps of the engineering workflows, the concept of guidelines and overwriting, the decision support, and the connection to other tools. The feedback during the proposed demonstration will be used to enhance Jarves’ underlying framework and its implementation regarding its effectiveness in guiding researchers through their research data management. Further tools will be integrated after initial feedback to provide a widely available and interoperable platform in the field of RDM in engineering. An extended evaluation will take place at a later time to validate the framework.
License: CC-BY-SA 4.0 ↗
Expressing science in knowledge graphs – SciMesh in the ELNs Kadi4Mat and JuliaBase - Presentation
Electronic lab notebooks (ELNs) are a fast growing topic in research data management (RDM), and for many scientists the entry point to RDM. However, there is not convergence to one ELN solution, on the contrary, more and more ELN products are being released. Since working in co-operations is very common in present-day scientific work, lack of interoperability between the ELNs is a hindrance for efficient collaboration. With SciMesh, we propose an approach that enables ELNs to share data. It is a schema that is able to map arbitrary scientific insights and workflows to a knowledge graph. The basic idea is to express causal relationships between single steps, so-called “processes”, in a directed acyclic graph. These steps typically represent either a manufacturing step of a specimen, or a measurement of that specimen. This way, cause and effect are both included in the graph, which expresses the scientific insight per se. We carefully added the fundamental building blocks that are necessary to allow for all demands of empirical science to be covered. Besides the “processes”, we introduce “concurrents” as an abstract concept to realise two important use cases: (a) processes that act on more than one specimen, and (b) processes that are divided into subprocesses. This way, SciMesh organises workflows along two axes, namely the time axis and the hierarchy axis. It is important to see that SciMesh is intentionally limited to metadata. Bulk data has to be stored elsewhere and is linked to from the SciMesh graph. An ELN can export data at any level of granularity (e.g. a single experiment, a sample, a sample series, a research project, or the complete database). The receiving ELN interprets as much of the SciMesh graph as it can. While visualising the time axis correctly is simple, the hierarchy axis can be a challenge. Therefore, SciMesh contains non-normative guidelines for how the nesting of processes should be interpreted. Typically, the receiving ELN will actively trigger the generation and transfer of the graph using the HTTP protocol. We discuss the different options for the authorisation against the sending ELN. We present a prototypical complete implementation of SciMesh in the ELN software JuliaBase. Additionally to sharing graphs for enriching the local view on the scientific data, JuliaBase also can export the data in RO-Crates that contain both the bulk data and the metadata in a SciMesh graph. This addresses use cases such as backup of the complete ELN, migration to another ELN solution, migration of parts of the data because a scientist moves to another institute, or analysing bulk and metadata outside the ELN. SciMesh handles gracefully merges and splits of specimens or samples. Furthermore, it makes it possible to refer to any intermediate state of a certain sample with one single URI. All relations in SciMesh point to the past, which enables validators to easily identify (and possibly disallow) additions to existing graphs that change causes retroactively. On the other hand, extending existing graphs with new research is encouraged and straightforward. At its core, the vocabulary of SciMesh is intentionally minimal. It is supplemented by terminology taken from existing vocabularies like schema.org, but we restrict this to truly generic domains like chemical formulae or units of measurement. We present a concept of compliance levels for applications that consume SciMesh graphs, where each level adds vocabulary that is required. At a certain grade of domain-specifiness, however, we will deliberately refrain from specifying any additional vocabulary and let the domain communities take over.
License: CC-BY-SA 4.0 ↗
The "ELN Finder" - a new service around Electronic Lab Notebooks - Tool demonstration
The new service "ELN Finder" supports the selection of a suitable electronic lab notebook (ELN) for specific usage scenarios. A common problem for researchers and RDM staff is to keep track of the large market of ELN software in order to select suitable ELN software or services for their own laboratory or institution. Up to now, there has been no continuously maintained and as complete as possible overview containing detailed information on the individual products, neither on a national nor on an international level. This gap is now closed by the "ELN Finder" (https://eln-finder.ulb.tu-darmstadt.de). The "ELN Finder" is thus a web service that helps in the selection of a suitable electronic laboratory book and significantly simplifies the otherwise very time-consuming search. The current version of the "ELN Finder" is as follows: By means of a sophisticated metadata scheme, more than 40 filter criteria are available, which in turn are divided into clearly arranged categories. The results list of the ELN tools identified is presented in an overview and contains brief descriptions of the individual tools. The "ELN Finder" currently contains detailed up-to-date information on 30 different ELN software systems. Editorial procedures have been developed and tested for further expansion of the database and continuous maintenance. The service is also intended to serve networking between users of the same system and to enable exchange of experience. The "ELN Finder" is organised by ZB MED - Information Centre Life Sciences, the editorial team consists of numerous experts and users of ELN tools from all over Germany. The technical implementation as a web service is carried out by the University and State Library (ULB) Darmstadt, based on the open source software DSpace7. The Tool Presentation introduces the new service and the editorial and technical processes behind it, describes the plans for the future and calls for editorial cooperation.
License: CC-BY-SA 4.0 ↗
Creating Data Management Plans with NFDI4Ing RDMO - Tool demonstration
Creating data management plans and fulfilling funders requirements for research data management is supported by RDMO for NFDI4Ing available at https://rdmo.nfdi4ing.de. The tool offers a structured interview for researchers guiding step-by-step through every aspect of the data life cycle. It provides an easy introduction to research data management and allows experienced data stewards to manage DMPs for larger collaborative projects. DMPs are a suitable means to ensure proper data management handling during research projects. In recent years funding agencies have raised requirements for data handling in funded projects with increasing frequency asking for data management plans to ensure FAIR data management. DMP tools are available to support researchers in the creation of such documents. The DMP tool and open source software Research Data Management Organiser (RDMO) is well established and available at many research institutions in Germany, but mostly offered as a discipline-agnostic tool. We adapted RDMO to more specifically address the needs faced in engineering. The step-by-step guide was developed as part of the NFDI4Ing Base Services measure Quality assurance in RDM processes and metrics for FAIR data (S-1). The development was supported by workshops conducted in the NFDI4Ing SIG Quality assurance & metrics for FAIR data. The interview is based on the DFG checklist Handling of Research Data [1] which is enriched with guidance and examples for researchers working in the engineering sciences. For DFG grant applications it is required to answer all these questions in the proposal and also for reporting. Regardless of a planned DFG submission, the checklist is compliant with the Science Europe core requirements for data management plans [2], therefore it can be used independently of a DFG proposal to create a DMP. The service is available for all interested researchers, login is provided via DFN-AAI or ORCID. RDMO is designed for collaborative work, it offers a simple rights management and allows project owners to invite members and set read and write permissions individually. Users can create project hierarchies, which is particularly interesting for data stewards of larger collaborative projects to manage DMPs of multiple projects. During the tool demonstration a general overview is given and new developments of the service are presented. Since going live in September 2022, more than 100 users have been using RDMO for their data management planning. For further improvement we are looking for use cases in the engineering community. [1] DFG (2021): Handling of research data. Checklist for planning and description of handling of research data in research projects, https://www.dfg.de/research_data/checklist [2] Science Europe (2021): Practical Guide to the International Alignment of Research Data Management - Extended Edition, DOI: https://doi.org/10.5281/zenodo.4915861
License: CC-BY-SA 4.0 ↗
Improved Ontology Curation and Visualization of SC3 Portal - Presentation
This paper describes improved curation, documentation, editing of ontologies in the SC3 Portal. Previous work [1] was mainly focused on ontology visualization methods namely, textual, graphical and hybrid to help users collaboratively develop ontologies. We evaluated the usability and effectiveness of the previous SC3 portal with a user survey and a case study involving real-world ontologies and domain experts. Based on users’ feedback from the survey we conducted, the portal was well-received by the community as a collaborative tool for ontology development. We addressed users' comments, suggestions, and we incorporated them in this version of the SC3 ontology curation portal. Collaboratively developing and curating ontologies is a challenging task that requires effective tools to support various stakeholders and tasks. The SC3 portal now facilitates collaborative ontology development and curation. It provides various visualization techniques, documentation generation, ontology comparison, and synchronization with standard version control systems, as well as organizing ontologies into collections and projects. The portal also provides user roles, role management and user management features. Roles define user’s access to a project and what administrator can add, delete or edit user’s rights to a project in the users management dashboard. The SC3 portal supports synchronization with standard Git-based version control systems such as GitHub and GitLab. Users are enabled to upload a specific version of an ontology from a Git-based repository, and switch to a newer or older version. The portal also enables the generation of on-the-fly documentation for ontologies. The portal integrates a wizard for documenting ontologies (WIDOCO) [3], a widely accepted open source tool, for generating documentation on-the-fly. We have also integrated ROBOT [2] within the portal. This tool compares any of two Git-based versions of an ontology. To edit ontologies the SC3 portal is using WebProtege. After making edits in an ontology in WebProtege the ontology can be committed from the portal to GitHub or GitLab repositories. To enhance user experience, we have extended the SC3 portal with several features. The portal offers collections and projects to maintain, document, and share ontologies. New users can request access to a project by sending email invitations directly from the portal to the project administrator. The project administrator can manage users and their roles within each project. References [1] F. Khan, F. Engel, N. Krdzavac, S. Auer, Collaborative and Cross-Stakeholder Ontology Engineering (short paper), In the proceedings of the International Workshop on Data-driven Resilience Research, 2022, Invited Contribution from the LSWT2023, Co-located with Data Week Leipzig 2022 (DATAWEEK 2022). Online: https://ceur-ws.org/Vol-3376/paper11.pdf [2] Jackson, R.C., Balhoff, J.P., Douglass, E., Harris, N.L., Mungall, C.J. and Overton, J.A., 2019. ROBOT: a tool for automating ontology workflows. BMC bioinformatics, 20, pp.1-10. [3] Garijo, D., 2017. WIDOCO: a wizard for documenting ontologies. In The Semantic Web–ISWC 2017: 16th International Semantic Web Conference, Vienna, Austria, October 21-25, 2017, Proceedings, Part II 16 (pp. 94-102). Springer International Publishing.
License: CC-BY-SA 4.0 ↗
Onto4OLAE: An ontology for the large area electronics domain. - Presentation
The data is the foundation for discovery and insight into the subject matter. Despite the enormous amount of data available, there are a number of issues that need to be addressed. These issues include 1) accessibility and reusability of data, 2) heterogeneity, 3) lack of relationships in information, 4) difficulty in getting the necessary information, and 5) semantic incompatibility. It is widely believed and reported that ontologies offer solutions to the above issues. This study focuses on building an ontology for a European project called MUSICODE and, generally, for the organic large-area electronics field. The Onto4OLAE was constructed following a seven-step procedure in web ontology language (OWL) and an open-source ontology editor called protégé. The bottom-up modeling approach was chosen to model all the identified applications for ontology. The data to construct a knowledge graph was collected using pre-defined data collection templates in Kadi4Mat. The developed conveniently represents structured and organized information and formally describes the OLAE domain with explicit ontological concepts and possible relationships between them. It is possible to model multiple distinct processes using Onto4OLAE, such as simulation, fabrication, and characterization. It illustrates knowledge about various manufactured devices and the materials required. Our study revealed that the present work is essential to show knowledge about organic electronics data, allows data integration, and brings intelligence to the OLAE system. Our findings also offer new insights into the OLAE domain, which have not been explored before. Nevertheless, Ontology development is an ongoing process, but the constructed ontologies can be well utilized to serve the purpose. The refinement takes place after regular intervals with the availability of new information. The ontology is published on GitHub pages and available via the following link. https://kit-mms.github.io/Onto4OLAE.github.io/
License: CC-BY-SA 4.0 ↗
Chart for the Core Objectives of Data Publishing within the Technical Infrastructure for Research Data Management - Poster Presentation
Academic data stewardship at universities has to fulfil many different roles. One of the most important is to build up a connection to researchers and bridge the gap between research data infrastructure and researchers. Different scientific communities have different requirements for research data management and expectations regarding text and data publication. In many of the sub-disciplines of engineering sciences, research data management practices are not established so far. When it comes to contact between data stewards and researchers, a common knowledge base has to be established to become clear about why and what actions for research data management has to take, that engineering researchers can adapt them for their research data management practices. Many research data management standards have to take into consideration. However, to this point of time, the research data management community lacks for data literacy graphics to explain how the technical data management ecosystem works together with standards like the findability, accessibility, interoperability and reuse (FAIR) principles. The chart is also aiming for establishing data practices in engineering sciences. Therefore, we developed a graphic for data literacy and consulting scenarios. The chart brings different research data management topics together like publication practices, semantic web, technical aspect of research data management repositories, third party researcher requirements on data and how these topics are connected and apply to the FAIR principles. Especially for engineering researchers, the different publication options and their purpose are unclear. Hence, the centre of the chart is an explanation of the three publications forms: article publication, data journal and data publication. Every of the forms work together with their own purpose. The classical scientific article describes research results and how they can be interpreted but does not have to describing the full structure and the abilities of the data set. This information gives a data journal article, what shows to hole data curation process complementary to the published data set. When it comes to data publication, the reason for several steps regarding data management are unclear for somebody who is not from the research data management community. Terms like “metadata” and their approaches are different in engineering and research data management (information science). Therefore, technical aspects of data publication and their connection to research data repositories will be shown. To show these connections, the system architecture of a research data repository will be described in a simple way. The FAIR principles refer to a couple of standards and protocols that have to be adapted by research data repositories. The standards will be described and how they are connected to the semantic web layers and what actions have to be taken by engineering researchers for a data publication to meet these standards. Furthermore, third party researchers have requirement to that data, which have to be adapted by the repositories, to make the data findable and reusable. These requirements will also be mapped by the chart.
License: CC-BY-SA 4.0 ↗
MaRDMO Plugin - Document and Retrieve Interdisciplinary Workflows Using the MaRDI Portal. - Presentation
Reproducibility plays a crucial role in the field of science, but there is a growing concern about the increasing number of reports regarding problems with reproducing scientific work [1,2]. These issues not only undermine the credibility of scientific research but also hinder the potential benefits that can arise from reusing and advancing existing scientific knowledge. The causes of reproducibility problems are diverse and encompass deliberate misconduct [3], as well as inadequate documentation of methodologies, software, parameters, conditions, and inconsistent naming conventions [2]. To address these challenges, the Mathematical Research Data Initiative (MaRDI [4]) has developed a standardized documentation scheme that allows researchers from different disciplines to summarize the relevant aspects of their interdisciplinary workflows, regardless of the research area or design (theoretical or experimental) [5]. This scheme serves as a means for scientists to document their interdisciplinary workflows and publish them on the MaRDI portal, a unique platform designed to house various mathematical research data. Moreover, researchers can access and retrieve these documentations through the corresponding Knowledge Graph (KG), enabling them to reproduce, develop, and re-document the original work in a reproducible manner. In order to facilitate the documentation and retrieval of interdisciplinary workflow documentations through the MaRDI portal, it is essential to provide a user-friendly and easily accessible platform. Ideally, this platform should seamlessly integrate into researchers' everyday work routines, without requiring them to directly access and manually interact with the MaRDI portal. The Research Data Management Organiser (RDMO) is an open-source web application that supports research data management. It offers discipline-specific question catalogues and allows users to generate data management plans. RDMO is currently utilized by numerous research institutions in Germany and has undergone testing in other European countries [6]. Its usage is expected to continue to grow in the future, particularly since most National Research Data Infrastructure consortia have endorsed its adoption [7]. As a result, RDMO has become an integral tool for many scientists across various research communities. Given RDMO's flexibility in allowing the design of customized question catalogues and additional functionalities, it presents an opportunity to develop a specific catalogue for documenting interdisciplinary workflows and establish a functionality that connects RDMO with the MaRDI portal. This integration would offer the desired low-threshold access to the portal. The MaRDMO plugin enables researchers to document and retrieve interdisciplinary workflows using the MaRDI Portal via RDMO. It consists of a questionnaire and an export/query functionality, accessible via the "MaRDI Export/Query" button in the project view. Further settings are configured through the question catalogue. The MaRDMO questionnaire is divided into seven sections, serving three purposes: documentation and retrieval of interdisciplinary workflows, as well as plugin settings. For documentation purposes, researchers must provide a general description, including the research objective, involved disciplines, and data streams. They should also state the underlying mathematical model, including its description, variables, parameters, and discretization. Additionally, researchers need to provide relevant process information such as process steps, algorithms, software, hardware, experimental devices, input and output data, and details regarding reproducibility. It is crucial to accompany all entries with identifiers, such as MaRDI identifiers, Wikidata QIDs, DOIs, and swmath IDs, to seamlessly integrate individual documentations into the existing research data landscape. For retrieval purposes, users define the entities to search for, such as research objectives, applied models, methods, and software. These entities can be searched using keywords or identifiers. The export/query functionality of MaRDMO gathers all the information provided in the questionnaire and allows for the creation or retrieval of documentations based on an entity search. When creating documentations, all the answers are integrated into MaRDI's predefined documentation scheme. Depending on the user's preferences, the completed scheme can be saved locally, previewed in the browser, or sent to the MaRDI portal to generate a wiki page. If the documentation is sent to the MaRDI portal, various components, such as the name, associated publication, research objective, model, methods, software, and input data, are integrated into the KG of MaRDI. To establish, for example, a connection between a new interdisciplinary workflow item and an associated publication, the following steps are performed: 1) Check if an item with a custom DOI already exists in the MaRDI KG. If it does, it is linked to the new interdisciplinary workflow item. 2) If no item with the custom DOI exists in the MaRDI KG check if an item with the custom DOI already exists in the Wikidata KG. If it does, it is integrated into the MaRDI KG and linked to the new interdisciplinary workflow item. 3) If an item with the custom DOI cannot be found in either KG, the full citation is retrieved from the DOI and ORCID. A new item is created in the MaRDI KG, and it is linked to the new interdisciplinary workflow item. The first two steps are repeated for the authors and journal. If no author or journal entries are found, they are created and linked to the new publication entry. To retrieve documentations, all the answers are translated into a SPARQL query. If relevant information is found in the MaRDI KG, the appropriate documentations are returned as wiki pages. MaRDMO is a plugin that empowers the entire research community to document and retrieve interdisciplinary workflows by utilizing the existing infrastructure provided by RDMO. Through the integration of documentations into the research data landscape, MaRDMO maximizes the network effects and offers scientists instant visibility. Simultaneously, the retrieval of documentations facilitates the convergence of new research insights from diverse disciplines. The straightforward structure of MaRDMO also allows for its application with other questionnaires and KGs to document and search for research objects beyond interdisciplinary workflows. [1] M. Baker, “1,500 scientists lift the lid on reproducibility,” Nature, vol. 533, pp. 452–454, May 2016. DOI: 10.1038/533452a. [2] Krishna Tiwari, Sarubini Kananathan, Matthew G. Roberts, Johannes P. Meyer, Moham- mad Umer Sharif Shohan, Ashley Xavier, Matthieu Maire, Ahmad Zyoud, Jinghao Men, Szeyi Ng, Tung V. N. Nguyen, Mihai Glont, Henning Hermjakob and Rahuman S. Malik- Sheriff, “Reproducibility in systems biology modelling,” Molecular Systems Biology, vol. 17, e9982, Feb. 2021. DOI: 10.15252/msb.20209982. [3] D. Fanelli, “How many scientists fabricate and falsify research? a systematic review and meta-analysis of survey data,” PLOS ONE, vol. 4, no. 5, e5738, May 2009. DOI: 10.1371/ journal.pone.0005738. [4] Christiane Görgen and Rainer Sinn, “Mathematik in der nationalen Forschungsdaten-infrastruktur,” Mitteilungen der Deutschen Mathematiker-Vereinigung, vol. 29, no. 3, pp. 122–123, Oct. 2021. DOI: 10.1515/dmvm-2021-0049. [5] Tobias Boege, Rene Fritze, Christiane Görgen, Jeroen Hanselman, Dorothea Iglezakis, Lars Kastner, Thomas Koprucki, Tabea Krause, Christoph Lehrenfeld, Silvia Polla, Marco Reidelbach, Christian Riedel, Jens Saak, Bj ̈orn Schembera, Karsten Tabelow and Marcus Weber, “Research-data management planning in the german mathematical community,” Nov. 2022. DOI: 10.48550/arXiv.2211.12071. [6] R. Arbeitsgemeinschaft. “RDMO - Cooperations.” (2023), [Online]. Available: hhttps:// rdmorganiser.github.io/en/cooperations/ (visited on 04/16/2023). [7] Harry Enke, Daniela Hausen, Christin Henzen, Gerald Jagusch, Celia Krause, Sabine Schönau, Lukas Weimer and Jürgen Windeck, “Data management planning: Concept for setting up a working group in the nfdi section common infrastructures,” Jan. 2023. DOI: 10.5281/zenodo.7540682.
License: CC-BY-SA 4.0 ↗
VocPopuli and Kadi4Mat for FAIR Data Collection in Experimental Sciences - Tutorial
Digitalization in experimental sciences is a process that can be seen as a strategy for harnessing rich data sets with the aim of finding more efficient solutions for investigating scientific outputs. One road to achieving full digitalization in research and development goes through the adoption of the FAIR data principles. FAIR is an acronym that stands for Findable, Accessible, Interoperable and Reusable data. Serially-produced FAIR data could be the key ingredient to enabling research results for scalable machine-learning-based analyses, and thus, it can potentially solve society’s greatest challenges. This tutorial will be shared between the groups of developers of the VocPopuli and Kadi4Mat software solutions. Rich, dynamic, and interoperable metadata are the foundation of any FAIR data infrastructure. However, there is hardly an established roadmap for the generation and curation of controlled vocabularies for scientific workflows. Therefore, for a few years now, our groups have been developing software solutions for the assembly of FAIR digital objects in research. One of the ways to approach the problems of digitalization is through the creation of controlled vocabularies, which we support via the software tool called VocPopuli. It enables the development of FAIR controlled vocabularies in a collaborative fashion. These vocabularies describe the experiments of interest for a given group, as well as all other equipment, processes, and data which pertain to them. Some of VocPopuli’s features are: (1) Login through a GitLab account, and user management through GitLab’s user right scopes; (2) The definition of contextual types such as procedure, experiment, equipment, data; (3) The creation of hierarchy of terms; (4) Each term can be defined through free text, synonyms, links to external or internal already existing terms; (5) For terms that describe quantities, special constraints on their ranges and units can be placed; (6) Multiple users can edit or comment on the terms collaboratively; (7) The final approval of the terms and their inclusion in the lab vocabulary is managed by a user with administrative rights; (8) The serialization of the vocabulary using SKOS and its publication; (9) The assignment of persistent URLs. Afterwards, the vocabularies can be used by the various other tools, which have been developed as part of the presented work. For example, FS-DigitalBook is a tablet-based application, which allows experimentalists to describe processes and objects relevant to their field of research in a FAIR fashion, without leaving the lab bench. The application utilizes the vocabularies developed with the help of VocPopuli as metadata schemata. These structures are used to create data input forms which can afterwards be filled with specific values, linked with files generated by the specific procedure at hand, and stored in an electronic lab notebook. All of VocPopuli’s and FS-DigitalBook’s functionality is brought to purpose because of the data that is generated with their help. For this we rely on the virtual research environment Kadi4Mat. Kadi4Mat is unique in its functionality to annotate the meaning of every piece of metadata with persistent identifiers. This is where the persistent URLs from VocPopuli and Helmholtz' PIDA service come to use. Kadi4Mat’s scheme of organizing digital representations of objects and processes into hierarchies and networks of records makes for a comprehensive research data infrastructure. These structures are utilized by one of Kadi4Mat’s newest feature, namely its ability to export metadata and descriptions as RDF serializations. RDF metadata serializations, together with all collected data files are then packaged and exported as interoperable RO-Crates. The RO-Crates can be then uploaded to any repository where they receive persistent identifiers, such as DOIs. Together this framework makes for a FAIR workflow where we collect all information directly at the source and lead it to its FAIR publication.
License: CC-BY-SA 4.0 ↗
esy.osm.topology - Tool demonstration
esy.osm.topology is a Python library which exposes heuristics to derive (energy) infrastructure topologies from OpenStreetMap (OSM). The OSM project maintains a public geo-dataset. In addition to street and routing information, many scientifically interesting infrastructure datasets like for example electricity, gas and telecommunication lines are available. However, as the primary use case of OSM is visualization, its geometries are not logically connected. Hence, OSM data has to be transformed into topologies, usually graphs, before it can be used as input data in models. This transformation is non-trivial, time-consuming, and highly dependent on the scientific context. With esy.osm.topology we propose a way to improve this status-quo. State-of-the-art tools and libraries that deal with infrastructure topology construction (e.g. OpenInfraMap, OSMnx, cityseer-api, noiseplanet, osmextract, osm-as-infrastrastructure, ...) are available, but they are either fragmented across many different programming languages or rely on database management systems or even external webservices. This leads to a high entry barrier, a sizeable setup and maintenance overhead or reproducibility issues. esy.osm.topology adds a degree of innovation by providing a “minimum-stack” software package, that doesn’t require elevated software privileges, constant maintenance or external services to be effectively usable. esy.osm.topology topology construction algorithms depend only on the core scientific computing libraries numpy and scipy, while OSM data extraction adds dependencies on protobuf, shapely and esy.osm.shape libraries. This small set of dependencies should allow straight-forward embedding of esy.osm.topology in larger tools or workflows. An example workflow using esy.osm.topology to construct an electricty grid from OSM data will be presented, detailing how: - Geometries are extracted from OSM data. - Heuristics are applied to aggregate geometries into topologies. - Resulting topologies can be visualized and inspected. Further more, we also discuss limitations and design trade-offs as well as technical details like computation time and memory usage of esy.osm.topology.
License: CC-BY-SA 4.0 ↗
How to teach good research data management to next generation researchers? - Presentation
Research work is now subject to comply with FAIR principles. Additionally, it is also subject to the practices of Open Science. Different stakeholders e.g. DFG are setting the goals of reproducible and repeatable research work. This not only requires the adequate handling of data but also the record of related information and practices during the research work. Therefore, different tools and workflows are being developed and suggested to achieve the goals of good research and its data management. Those tools and workflows facilitate researchers and ease the research management tasks e.g. by the means of standardisation, automation of processes and record of corresponding information. The researches of now a days are interdisciplinary and work collaboratively where participants are located at distinct locations, belongs to different domains and have different levels of competencies. In such cases, provision of tools and specification of workflows is not enough. Just like other management, good research data management is a skill that need to be taught to the researchers in a systematic and detailed way. So that they could make right decisions where and when needed. As a result, the contents and the materials for the education of good research data management become important. In this presentation, mainly approaches to deliver good RDM practices will be presented. We will be presenting our defined approaches and experiences based on our work done during the course of TRR277 AMC (a DFG funded CRC consisting over 120 researchers that are still growing). We will be presenting the lists of common skills and understanding that every researcher should know before and during the course of research work on one hand. On the other hand, skills and knowledge relating to the common aspects of RDM systems will be addressed. Additionally, contents and methodologies while considering available means of communication both in person and online to increase acceptance and understanding will be presented. In conclusion, the skills which relates to the pre-research learning and practices relating to good RDM will be emphasised. Therefore, such contents could be suggested for teaching to the future researchers and data stewards during the regular academic discourse. The presented contents and strategies may also be adopted for small projects to large scale collaborative research centre projects.
License: CC-BY-SA 4.0 ↗
BenchForge: a tool for benchmarking heterogeneous engineering software - Presentation
In the industrial context, engineering software is a tool for improving physical product designs regarding functionality and efficiency. Although engineering software is used in product design “as is”; the software changes as new versions are released. In order to guarantee the trustworthiness of new versions, it is necessary to evaluate the results using benchmark tests objectively. In an industrial context, emphasis is not only on quantification of results, but also on execution speed, and any alterations in functionality between versions must be monitored. In larger companies, various engineering software are used to design closely related product components. A comparison between the performance of engineering software is highly beneficial for saving costs and making informed decisions about future software acquisition. To facilitate benchmark comparisons between different software and software versions, we have developed BenchForge. This Python tool performs semi-automated regression tests, parameter studies of heterogeneous engineering software, and archives and compares tabulated result data. Engineering product design typically requires HPC clusters, but BenchForge is not limited to these platforms. Outsourcing the software-specific control into a shell-run script achieves interoperability between heterogeneous engineering software. Thereby, any simulation software package accessible through shell scripts can be controlled by BenchForge. On the data output side, the broadly supported comma-separated value (CSV) format is chosen as a basis for interoperable data exchange. An optional post-processing script can convert data if the CSV format is not natively supported. Focus is placed on result comparison, reproducibility, and simplicity of use for engineers. Execution of three scripts completely handles the testing stages of setting up and executing a test case set, collecting data from the test case set and storing it in an archive, and comparing data sets from different runs, e.g., with different software versions or different software. The automation allows to setup/run of one or multiple cases. The system scheduler handles license management responsibility. Within this talk, we demonstrate the application of BenchForge to the comparison of several well-known Computational Fluid Dynamic Tools, such as ANSYS Fluent, StartCCM+, OpenFOAM, and PreonLab.
License: CC-BY-SA 4.0 ↗
Building a Data Transfer Federation between Research Centers - Presentation
Building a Data Transfer Federation between Research Centers Mozhdeh Farhadi*, Serge Sushkov*, Andreas Petzold* *Karlsruher Institut für Technologie Cross-site collaboration between research centers is becoming increasingly crucial, with researchers accessing storage or computing resources from other institutions. However, this collaboration results in the need for large data transfers between different storage systems for computation or archival purposes, which can be a challenging and time-consuming task. To address this need, the Steinbuch Centre for Computing (SCC) at Karlsruhe Institute of Technology (KIT) designed a data transfer federation in the context of NFDI4Ing project, base services-S4:repositories & storage. A data transfer federation is a collaboration of storage providers across multiple organizations, to enable access to data with federated identities and automated, scalable data access and data transfers between the storage systems. Our proposed federation allows researchers to access and transfer data between different storage systems using their home organization's user account, based on their access rights to the resources in a collaborative project. The storage systems in a federation are either dedicated large-scale systems or systems associated with High-Performance Computing (HPC). At SCC, we have integrated our Large Scale Data Facility: Online Storage (LSDF OS) [1] with WebDAV [2] protocol and OAuth2 [3] authentication to enable access of third-party applications to the storage service. Beyond improved support for programmatic access using OAuth2, the WebDAV server enables users to inspect the LSDF OS filesystem via their web browser. For enabling data movement, we have deployed an instance of the File Transfer Service (FTS) [4] on-premises and integrated it with our identity provider (IdP). FTS is a low-level data management service that orchestrates reliable bulk transfer of files from one storage endpoint to another. It is an open-source software developed by CERN that distributes the majority of the Large Hadron Collider (LHC) data across the Worldwide LHC Computing Grid (WLCG) infrastructure. However, FTS is designed to be used with one identity provider, whereas in a federation, more than one IdP is involved. To address this limitation, we have designed a central IdP to issue tokens that are recognizable by the downstream identity providers which manage users’ access to their corresponding storage service in the federation. At each storage service, tokens issued by the central IdP are resolved to the corresponding user identity at the local IdP via a mapping policy. To achieve this, a unified approach in the federation regarding the information included in the token is necessary. In conclusion, the data transfer federation enables users to have seamless access to a wider range of resources, thereby simplifying collaboration between research centers. [1] https://www.scc.kit.edu/forschung/11843.php [2] https://www.ietf.org/rfc/rfc4918.txt [3] https://datatracker.ietf.org/doc/html/rfc6749 [4] A. A. Ayllon et al, FTS3: New Data Movement Service For WLCG, J. Phys.: Conf. Ser. 513 032081 (2014)
License: CC-BY-SA 4.0 ↗
Clean Code Principles for Scientists: Enhancing Code Quality and Collaboration - Workshop
In the scientific community, producing high-quality and maintainable code is crucial for accelerating research progress and facilitating collaboration. Clean code enhances readability, making scientific software more easy to understand and reuse by peers and can therefore play a key role in producing FAIR research software. By following clean code principles such as modularization and code organization, code becomes more accessible and reusable, enabling scientists to build upon existing work and share their code with others. Furthermore, clean code enhances maintainability, making it easier to update and modify code to adapt to changing research requirements. By embracing clean code, scientists can create software that is in line with the FAIR principles, fostering reproducibility, collaboration, and the advancement of scientific knowledge. The workshop will begin by providing an overview of the significance of clean code in scientific research, with a particular focus on the advantages it offers in terms of code quality, productivity, and collaboration. Participants will learn how clean code principles align with the unique challenges faced by scientists, such as changing requirements and the need for reproducibility. Throughout the workshop, participants will learn about the core concepts of clean code, covering topics such as naming conventions, code organization, and effective commenting. Practical exercises and examples will reinforce these principles, allowing participants to experience firsthand the impact of clean code on readability and maintainability. The workshop will emphasize the importance of refactoring techniques, teaching participants how to improve existing code without altering its functionality. Participants will explore code smells and anti-patterns commonly encountered in scientific codebases, and learn appropriate refactoring strategies to address them. Through hands-on exercises, participants will gain experience in extracting methods, reducing code duplication, and simplifying complex code. By the end of this workshop, participants will have understand the core principles of clean code and learned essential techniques for improving code quality. They will also have acquired basic skills to create high-quality code that is easy to maintain. Join us for this workshop and unlock the potential of clean code principles. The only way to go fast is to go clean!
License: CC-BY-SA 4.0 ↗
Workshop on FAIR Metrics in Engineering Sciences - Workshop
Making digital objects FAIR (findable, accessible, interoperable, reusable) is one of the cornerstones for transparency of research data and supporting their reusability. The FAIR principles are designed as high-level guidance, which needs to be interpreted and implemented in concrete FAIR metrics, e. g. domain- or object-specific. While several metrics have already been developed – including general ones or ones for software –, none of them are currently specifically for the engineering sciences. This workshop aims at considering FAIR principles from the engineering science domain point of view. The workshop will start with a general introduction and explanation of the fifteen FAIR guiding principles. Based on that, workshop participants will be invited to share what FAIR means to them in their research activities and how they implement it. General and engineering-specific elements of FAIR will be distinguished during this part. In addition, participants will be asked to categorize the importance of each principle for them. This part of the session will be conducted on an interactive whiteboard so that everyone can actively participate. It primarily addresses researchers in the engineering sciences and data stewards working together with engineers, while librarians and IT staff is highly welcome as well to share their perspective. The workshop will end with the question whether an engineering-specific FAIR metric is required and how it could be bounded. The participants will be asked to give their opinion on that based on the FAIR elements identified before, even when a structured comparison to existing FAIR metrics is not part of this workshop. The workshop outcome will be used to figure out whether engineering sciences need a FAIR metrics on their own and – if yes – how this could be designed. An individual metric might provide the benefit to be better applicable compared to a general one but might include the risk of loosing comparability to existing metrics. After the workshop, the results will be evaluated and documented in a publication. Depending on the workshop outcome, a potential FAIR metric for the engineering sciences would be developed in the NFDI4Ing work package S-1-4 and made publicly available. Overall, this workshop supports NFDI4Ing’s goal to “develop, disseminate, standardise and provide methods and services to make engineering research data FAIR” [https://nfdi4ing.de/about-us/].
License: CC-BY-SA 4.0 ↗
"FAIR-by-Design" Artifacts: Enriching Publications and Software with FAIR Scientific Information at the Time of Creation - Presentation
In several research disciplines, the use and development of software have become an integral part, with researchers reporting in publications the results obtained with software and concepts implemented in software. Consequently, publications and software have become two core artifacts in academia with increasing importance for measuring research impact and reputation. The research community has made great efforts to improve digital access to publications and software. However, even now that these artifacts are available in digital form, researchers still encapsulate the scientific information in static and relatively unstructured documents unsuitable for communication. The next step in the digital transformation of scholarly communication requires a more flexible, fine-grained, context-sensitive, and semantic representation of scientific information to be understandable, processable, and usable by humans and machines. Researchers need support in the form of infrastructures, services, and tools to organize FAIR scientific information from publications and software. Several research disciplines work on initiatives to organize scientific information, e.g., machine learning with “Papers-with-Code”, invasion biology with “Hi-Knowledge”, and biodiversity with “OpenBiodiv”. However, these initiatives are often technically diverse and limited to the respective application domain. For this reason, we from the task area Ellen of NFDI4Ing (and in collaboration with NFDI4DataScience and NFDI4Energy) decided to use the Open Research Knowledge Graph (ORKG), an innovative infrastructure for organizing scientific information from publications and software. The ORKG is a cross-discipline research knowledge graph that offers all research communities an easy-to-use and sustainably governed infrastructure. This infrastructure implements best practices, such as FAIR principles and versioning, with services combining manual crowd-sourcing and (semi-)automated approaches to support researchers in producing, curating, processing, and (re-)using FAIR scientific information from publications and software. As a result, organized scientific information is openly available in the long term and can be understood, processed, and used by humans and machines. Thus, research communities can constantly build, publish, maintain, (re-)use, update, and expand organized scientific information in a long-term and collaborative manner. While the ORKG currently focuses on organizing scientific information from published publications and software, we aim to help researchers create “FAIR-by-Design” artifacts to improve their storage, access, and (re-)use, using the ORKG as exemplary infrastructure. The idea of “FAIR-by-Design” artifacts is that the creators of an artifact describe it with extensive and FAIR information once and in parallel to the time of creation. This FAIR information is embedded directly into the artifact to be available to anyone at any time. Specifically, we developed two tools (SciKGTeX for publications and DataDesc for software) that support researchers in the role of author and developer to enrich their publications and software at the time of writing and development with FAIR scientific information embedded into the respective artifact. SciKGTeX is a LaTeX package to annotate research contributions directly in LaTeX source code. Authors can enrich their publications with structured, machine-actionable, and FAIR scientific information about their research contributions. SciKGTeX embeds the annotated contribution data into the PDF’s XMP metadata so that the FAIR scientific information persists for the lifetime of the artifact. DataDesc is a toolkit that combines different tools to describe software with machine-actionable metadata. Developers can describe Python software and its interfaces with extensive metadata by annotating individual classes and functions directly within the source code. DataDesc converts all metadata into an OpenAPI-compliant YAML file, which various tools can render and process. Regarding the research data management (RDM) lifecycle, both tools target the production phase to support researchers in creating“FAIR-by-Design” artifacts. Creating “FAIR-by-Design” artifacts helps to improve their storage, leading to better access to artifacts and thus laying the foundation for their effective (re-)use. Using the ORKG as exemplary infrastructure, we demonstrate with two proof-of-concepts how infrastructure providers can use the artifacts from SciKGTeX and DataDesc to store the FAIR scientific information in their systems. In the case of SciKGTeX, the ORKG recently added a new upload feature for SciKGTeX annotated PDFs to allow researchers to add the FAIR scientific information of publications quickly and easily. In addition, the ing.grid journal provides a version of their LaTeX template that integrates the SciKGTeX. For DataDesc, we plan such an upload feature and similar use by the community in future work. Researchers only need to create a “FAIR-by-Design” artifact once, and can reuse it on multiple infrastructures to improve their dissemination and discoverability. With improved storage, researchers can more easily discover and access publications and software to determine whether an artifact fulfills their information needs. However, researchers do not have to rely on such infrastructures to find, access, and assess publications or software. When they encounter a “FAIR-by-Design” artifact, it embeds the additional information itself so that they can review the artifact themselves with the same information base. Improved discoverability and accessibility lay the foundation for effective (re-)use as researchers can better understand an artifact. In the case of the ORKG, we can even (re-)use the information from SciKGTeX and DataDesc stored in the ORKG interchangeably. A publication annotated with SciKGTeX can reference a software annotated with DataDesc stored in the ORKG and vice versa. Overall, enabling researchers to create “FAIR-by-Design” artifacts is a promising approach to support the downstream phases of storage, access, and (re-)use in the RDM lifecycle. In our presentation, we want to explain the idea of “FAIR-by-Design” artifacts in more detail using concrete examples based on the two tools and in combination with the ORKG. We believe that the idea of “FAIR-by-Design” artifacts is of interest to the research community. The two tools can inspire other researchers to extend our original approaches and develop new ones to create more “FAIR-by-Design” artifacts by enriching artifacts with FAIR scientific knowledge at the time of creation. Furthermore, we hope to encourage and motivate researchers to use our tools more intensively and thus establish them. In particular, the existing and planned future integration with ORKG and the existing collaboration with the ing.grid journal are motivating incentives for researchers to use SciKGTeX and DataDesc actively.
License: CC-BY-SA 4.0 ↗
SkoHub – Unleashing the potential of controlled vocabularies - Presentation
Controlled vocabularies, authority data, and other "Knowledge Organization Systems" (KOS) have long been a central part of knowledge organization in libraries, research and education. They are widely used in different domains supporting the discovery of resources by connecting them to topics. The SkoHub ecosystem, designed and developed by North Rhine-Westphalian Library Service Centre (hbz), comprises a growing set of software modules to get optimal benefit from your controlled vocabularies by making use of SKOS (Simple Knowledge Organization System) and other web standards. The core module - SkoHub Vocabs - enables the lightweight publication of knowledge organization systems as Linked Open Data based on SKOS. This facilitates, for example, compliance with FAIR Data principles in Digital Humanities projects or in research data management. SkoHub Vocabs is being used by various stakeholders from a educational, scholarly or library contexts. The module SkoHub Reconcile builds on the work done to develop a web API that data providers can expose, for easing the reconciliation of third-party data to their own identifiers. SkoHub Reconcile is currently under development but already quite mature, supports the simple reconciliation of data with a controlled vocabulary, e.g. by using the widely used software OpenRefine. Use cases include enrichment of less structured data with authoritative identifiers and additional data or entity linking. The SkoHub PubSub module supports a novel approach to knowledge organization systems by turning them into communication hubs for publishers and information seekers. Topics in the form of SKOS concepts become actors in the Fediverse that I one can follow - for example, on the rising microblogging service Mastodon. As soon as new content is published on that topic, I'm notified via a push notification. SkoHub thus seeks to support forms of self-organized social exchange based on recognized web standards and could support in the communication of scientific communities in the future. SkoHub PubSub has been successfully tested in a proof of concept and will be further developed in the future. The hbz has a long track record of providing reliable open metadata infrastructure for libraries and other interested parties. We take over the maintainer duties for SkoHub development and invite engagement by the community of SkoHub users, e.g. by providing bug reports, feature requests or by improving the documentation. To enable community-based development, all SkoHub software is provided under an open license and the development happens in a transparent way on GitHub (see the CONTRIBUTING.md at https://github.com/skohub-io/skohub-vocabs/blob/main/CONTRIBUTING.md and the Kanban board at https://github.com/orgs/skohub-io/projects/4/views/1). The presentation will introduce the SkoHub software ecosystem and illustrate its possibilities with practical use cases from the fields of libraries, research, education and standardization.
License: CC-BY-SA 4.0 ↗
Strengths and deficits of the CEN Workshop Agreements for data documentation in materials modelling and characterization - Presentation
Within materials modelling and characterization, European initiatives, including Horizon projects related to the European Materials Modelling Council (EMMC) and the European Materials Characterization Council (EMCC), have been working toward standardized data and metadata documentation, which resulted in a series of metadata standards and procedural recommendations that were accepted and published as CEN workshop agreements (CWAs): CWA 17284 for model data (MODA), CWA 17815 for characterization data (CHADA), and CWA 17960 for model-topology graphs (ModGra). The present contribution explores the outcome and uptake of these standardization efforts. It discusses the shortcomings that will become limiting factors to the uptake of CHADA and MODA if it is ever seriously tried to disseminate them beyond the very core of the community they originate from. CWA 17284 MODA first and foremost is a set of tables or forms that a user is supposed to fill in with plain text content. The standardization process for MODA was based on a previous community surveying exercise, the Review of Materials Modelling (RoMM). RoMM and MODA successfully accomplished something very important: They defined basic (fundamental) entities and provided a shared conceptualization of materials modelling that has since been in use within the EMMC community, across research groups that had not previously shared a common terminology and perspective. As a more problematic outcome of this process, however, MODA is by construction narrowly limited to a static set of physical equations; theoretical or methodological work that goes beyond these pre-established categories either needs to be artificially mapped to the one that seems closest, or it cannot be accommodated at all. In addition to the tables, work toward providing a graphical notation for provenance in molecular and multiscale modelling has led to MODA graphs, which are part of the CWA standard. The MODA graphs are related to the MODA tables in that some of the sections can show up as nodes; however, this is not done consistently, and data/information entities often occur as nodes instead. Where that is the case, it is hard to see how the MODA tables and graphs relate to each other conceptually at all. RoMM includes a corpus of MODA based simulation workflow documentations from projects which were by EC policy required to supply such material; overall, this material mainly shows that the notational elements (arrows, nodes, etc.) are highly ambiguous and that there even are contradictory opinions among users as regards their purpose and meaning. CWA 17815 CHADA follows the same approach as MODA, and it has the same structure; the difference between the two is only that CHADA is supposed to be used for documenting experimental data provenance and experimental workflows, whereas MODA is to be used for modelling and simulation data provenance and workflows. Same as for MODA, the result is a human-intelligible, but often highly ambiguous representation that will usually tell the reader less than looking into a paper that describes how the experiments were conducted. These two CWAs have never been in use outside the core community consisting of projects where they were made mandatory by the EC and its responsible project officers. Within that core community, the assessment of this approach has become more and more sceptical. It will be unavoidable to go beyond CHADA/MODA. Their limitations include an idiosyncratic arrangement of data items that makes it hard to align them with other semantic artefacts, including the EMMO foundational ontology. However, the most salient issue surrounding CHADA/MODA is that researchers are expected to go great lengths to document their research outcomes' provenance in detail, but despite that, the documentation does not become meaningfully machine-actionable. CWA 17960 ModGra promises to deliver the required paradigm shift, replacing MODA with a more flexible and meaningful way of denoting how physical quantities at multiple levels relate to each other, and how one simulation step can feed into another. ModGra is the community-agreed version of a notation developed over years, with a variety of use cases in computer aided process engineering. The core concept in ModGra is that of the process model topology, defined in analogy with Petri nets as a directed graph of nodes connected by directed arcs. The nodes are token capacities, and the edges are arcs transporting tokens. Different sorts of nodes can contain different sorts of tokens: In the case of a control capacity, representing the logics of the simulation workflow, the tokens are information items, whereas the tokens contained by physical capacities are extensive physical quantities, representing the logics of the model of a physical system. [The abstract text above is an excerpt from an upcoming publication; see also the preprint under doi:10.5281/zenodo.7867976.]
License: CC-BY-SA 4.0 ↗
Using a new HPMC sub-ontology within the Metadata4Ing framework: classification and extraction of data for engineering applications - Presentation
An ontology defines a common vocabulary and describes the syntactic as well as the semantic interoperability, including machine-interpretable definitions of basic concepts in the domain and the relations among them. The NFDI4Ing consortium has developed the ontology Metadata4Ing [https://git.rwth-aachen.de/nfdi4ing/metadata4ing/metadata4ing] for a process-based description of research activities and their results as a common classification of engineering data in a taxonomic hierarchy with standardized vocabulary and procedures. In engineering disciplines using high performance computing systems (e.g. Computational Fluid Mechanics, CFD), there is no consistent and established terminology yet, neither a standard to describe data sets in a fully semantic way. This is caused by the (often) non-existent awareness for the benefits of FAIR data sets, the lack of knowledge on how to implement ontologies in everyday research and the lack of tools supporting this process. In order to establish a consistent terminology for CFD workflows in high-performance computing (HPC) systems, a community based HPC-sub-ontology is being developed and improved within the framework of Metadata4Ing. The subordinate classes and relations of Metadata4Ing are built according to the principles of inheritance and modularity. Inheritance describes how a subclass inherits all properties of its superordinate class, possibly adding some new ones. Modularity describes the independence of all expansions of each other; this enables for instance to generate expanded ontologies for any possible combinations of method × object of research [https://zenodo.org/record/5957104#.ZHCTV3xByUk]. The expansion to a HPC-sub-ontology is based on the modularity and fits in the primarily Metadata4Ing classes of method, tool, object of research. The expansion includes suggestions of unambiguous, well-documented terms for domain related metadata expressed in classes, object properties (relations) and data properties. These classes have been developed in a community-based approach and represent common methods and tools for workflows in engineering research on HPC systems. The terminology and definitions are quoted from established vocabularies as schema.org, w3.org, DCMI Metadata Terms and QUDT or newly determined and documented within the ontology file. This semantic HPC-metadata-set can easily be adapted to individual needs and allows for the expansion to additional terminologies describing new workflows and investigated domains. The use of the Metada4Ing ontology and the HPC expansion generates a data description in a single knowledge graph approaching the fulfilment of FAIR data principles for HPC metadata. Especially the findability and interoperability of datasets is going to be substantially improved by a consistent and unambiguous terminology with semantic relations and restrictions. An overview of Metadata4Ing is available at the ontology documentation [Link]. The ontology code is developed at m4i's GitLab repository [Link], where a special branch for the HPC expansion is publically available and open for contributions, comments and suggestions. To give an example of the usage of the new HPC sub-ontology, metadata will be extracted from both numerical and experimental datasets related to fluid-dynamics problems and classified according to the newly proposed ontology scheme. An improved version of HOMER (the metadata crawler developed at TUM, https://gitlab.lrz.de/nfdi4ing/crawler/), will be employed to read out the Metadata4Ing ontology together with the newly-developed sub-ontology and retrieve metadata from two CFD studies, performed with the in-house code ALPACA, the semi-commercial solver NSMB and from an experimental wind-tunnel-test dataset. A future step will be the coverage of the complete data life cycle in a holistic approach, by providing the possibility to preserve and publish or share the extracted metadata along with the research dataset. Therefore, an editable and fillable front-end of the ontologies classes and data properties will be displayed in a repository (e.g. Coscine by RWTH) to match with the extracted metadata and enable the metadata publication in a findable, accessible and interoperable manner.
License: CC-BY-SA 4.0 ↗
Text and Data Mining for Research Purposes - Presentation
Text and Data Mining (TDM) is an established scientific method. In addition to other types of research data, especially scientific publications may be an essential element of the text corpus for TDM or training material for AI. The print publications are accessible in the reading rooms of libraries and access to licensed digital databases and journal packages is provided by libraries. In the summer of 2021 the statutory exception for TDM in German copyright law was adapted to the requirements of the DSM-Guidelines of the EU. What do the new and revised statutory exceptions allow exactly? May researchers use the print or digital resources of libraries for TDM? May digital resources of publishers be used, especially if they are located outside EU? Within the work package S-7-2 of Base Service Measure S7 of NFDI4Ing, „Guidelines for Text and Data Mining for Research Purposes in Germany“ were developed which describe details of the new and revised statutory exceptions for TDM in German copyright law with regard to scientific publications. The guidelines were published in Q4/2022 (https://oa.tib.eu/renate/handle/123456789/10352) and the results are presented here.
License: CC-BY-SA 4.0 ↗
The Sandbox Concept for Ontology Management - Presentation
This paper presents the Sandbox concept for ontology management. It is a collection of synchronized services within NFDI4Ing terminology service. The actual terminology services, such as the Ontology Lookup Service (OLS) [3], require unification and standardization of ontologies throughout their life-cycles for efficient ontology management. These services do not support ontology versioning, quality assessment, pairwise similarity between a pair of ontologies, computing, searching and curating mappings between ontologies, and semantically searchable ontology history services. All these features are important from the aspect of an ontology collaborative development, maintenance, reuse, and application. So far, however, the OLS based NFDI4Ing terminology service has not directly dealt with these aspects. With the support of various project partners (also outside the NFDI), we are therefore currently working on the Sandbox construct that enables us to make new features available to the community for review and feedback at an early stage. Depending on acceptance and maturity, the new ontology management services can then be transferred to the productive system. These services rely on the REST API of NFDI4ING terminology service for retrieving essential ontology information. Services also provide another REST API and user interfaces that feature the respective ontology management tasks. The four main tools implemented as part of the Sandbox concept are History Management, computing and visualizing mappings between ontologies, Quality Assessment and Pairwise similarity services respectively explained in the following paragraphs. History Management (HM) service enables analysis of semantic differences between ontologies. The HM service collects information managed by a Git-based versioning system. In the next step the service builds a chain of connected parent-child commits. Afterwards, the data are processed by the ROBOT tool [2] to find changes that have been made in ontology. For example, the robot tool finds changes in annotation or sub class of assertions. As a result, the service generates a timeline of changes that could be processed as the history of the whole ontology or could also be narrowed down to track development of a particular attribute. Ontology matching is a problem of automatically computing mappings between ontologies [1]. We use LogMap [1] ontology matching tool to compute mappings between ontologies ingested in NFDI4Ing terminology service. We store results of the mapping computation in JSON format. It contains information about number of mappings, number of conflictive mappings, a list of mappings and conflictive mappings, including confidence and structural confidence rates for each mapping, mapping direction, and mapping type between ontology ingredients such as classes, properties, and individuals. Data stored in JSON format are visualized in tables and graphs. Quality Assessment service is gathering Github or Gitlab based version control data related to ontologies, developers, researchers. Users can make informed decisions about which ontologies to adopt, contribute to, or collaborate on. It fosters community engagement, quality improvement, and ensures legal compliance and accessibility. Furthermore, the availability of documentation, readme files, and releases enhances the ontology's usability, understanding, and reproducibility, enabling efficient and effective utilization within research, development, and knowledge sharing endeavors. Pairwise Similarity (PS) service computes percentage of shared characteristics between selected ontologies. A total number of elements and a number of shared characteristics are computed based on selected ontologies. These numbers are used to compute the percentage of share characteristics. For example, two selected ontologies can share 7.62% of namespaces as their characteristic. Percentages of shared characteristics along with all characteristics and its elements are presented in a chart. The Sandbox concept itself, as well as the presentation of a selection of its tools, are the core topic of this contribution. These Sandbox’s services are currently under development and will gradually be transferred to the Sandbox and published once their current development versions are baselined for production. References [1] Jiménez-Ruiz, E. and Cuenca Grau, B., 2011. Logmap: Logic-based and scalable ontology matching. In The Semantic Web–ISWC 2011: 10th International Semantic Web Conference, Bonn, Germany, October 23-27, 2011, Proceedings, Part I 10 (pp. 273-288). Springer Berlin Heidelberg. [2] Jackson, R.C., Balhoff, J.P., Douglass, E., Harris, N.L., Mungall, C.J. and Overton, J.A., 2019. ROBOT: a tool for automating ontology workflows. BMC bioinformatics, 20, pp.1-10. [3] Jupp, S., Burdett, T., Leroy, C. and Parkinson, H.E., 2015. A new Ontology Lookup Service at EMBL-EBI. SWAT4LS, 2, pp.118-119.
License: CC-BY-SA 4.0 ↗
Coscine - Makes research data FAIR! - Tool demonstration
A large proportion of researchers only become involved with Research Data Management (RDM) and the associated FAIR-principles shortly before publication. However, this usually involves a huge amount of additional work, compared to if the researchers had already paid attention to compliance with the FAIR-principles and appropriate handling of metadata from the planning stage of the project onwards. In order to avoid loss of research data (and the associated metadata) and to enable subsequent use of the data even after the end of the project, detailed Research Data Management is of crucial importance.
To support researchers, the open-source platform Coscine helps them both store and manage the research data. Additionally, it offers a solution to archive the data after completing project. In order to ensure compliance with FAIR-principles in accordance with Good Scientific Practice, the associated metadata is stored with the research data. The users of the platform are thus supported from the planning of the project through to archiving (along the research data lifecycle).
A registration in Coscine is possible via an institutional account as well as ORCiD. This allows collaborations within a research group as well as the participation of external partners in a project. In addition, different roles can be assigned within a project (owner, member and guest), each of which has corresponding read and write rights. The clearly defined project structure in Coscine, consisting of main projects and associated sub-projects, enables simple data management. Metadata (e.g. project name, description, discipline, participating organizations, etc.) is already entered during the creation of the respective projects. At the project- and resource level, metadata entry is mandatory in Coscine and is allowed in various schemas (RDF, OWL and SHACL standards). If the project settings do not contain visibility restrictions, the entered metadata can be searched publicly and shared within Coscine.
For each project, different data sources, so-called resources, can be created in Coscine, which are uniquely and permanently identifiable by an ePIC PID. In the selection of resources, Coscine currently offers, among other solutions, access to the Research Data Storage (RDS), which is a consortially operated object storage system. The storage system is funded by the Ministerium für Kultur und Wissenschaft des Landes Nordrhein-Westfalen and the Deutsche Forschungsgesellschaft. The RDS can be used both via the web interface (RDS-Web) and via so-called S3 clients as an interface to the RDS-S3 storage. For research data with a high required level of data protection, the RDS-WORM resource can also be used. The use of RDS resources is currently only possible for participating universities but will be available for universities, technical colleges, and research institutions throughout North Rhine-Westphalia in the close future. Alternatively, GitLab or data stored in other directories (so called Linked Data) can also be linked in the resource selection, so that researchers from non-participating universities of the RDS can already use the platform. Furthermore, Coscine is connected with the application profile generator AIMS, so that individual and project-specific profiles can be created and research data is made findable and interoperable.
After completion of a project, the research data (and metadata) can be archived in Coscine. Good scientific practice, which provides archiving for ten years after completion of the project, serves as a guideline here.
The planned tool demonstration of Coscine will cover a brief introduction of the platform including the features as well as the benefits for researchers in terms of compliance with Good Scientific Practice and the FAIR-principles. This is followed by a demonstration of the platform interface and a step-by-step guide through the registration process, creating a project, inviting members to join a project, and creating a resource. As each step is taken, it is also shown how to add appropriate metadata and how to meet the needs of the individual researcher by creating an individual application profile. After the tool demonstration, the audience should have gained a general insight into how the platform works.
The demonstration takes into consideration that Coscine is one of the first Services that are connected to Jarves. Therefore, the demonstration shall not only demonstrate the functionalities and new features of Coscine itself, but also enable users of Jarves to better use the toolchain of RDMO, Jarves and Coscine.
License: CC-BY-SA 4.0 ↗
Semantic Modeling for FAIR Data using PLASMA - Tool demonstration
Although the FAIR (findable, accessible, interoperable and reusable) principles have essentially become a central element of (research) data management, many data owners are faced with the challenge of providing their data in a way that satisfies those requirements. Especially in engineering, where, for example, many individual data streams are recorded on the factory floor by different systems and sensors in different processes, data management in accordance with FAIR principles plays an important role. In the context of, e.g., machine data, this requires to provide sufficient context information alongside the raw data values so that other engineers, data scientists and even other machines are able to properly interpret and process the data. Even on very simple examples, such as a single sensor reading from a PLC controller, missing context can render obtained values partially unusable if reference points, units of measurement or system settings are not provided. In other words, if we want to share data with other parties, we have to be able to define this additional contextual information. Over the last few years, the use of semantic technologies, which are also one of the core components of data spaces, has become increasingly important. By using shared conceptualizations, such as ontologies, data attributes of datasets, e.g., columns in a table based format, are mapped to classes of such an ontology. By creating such mappings (semantic labeling), we become able to interpret the data (attributes) of one data set in the scope of the ontology. By additionally establishing context in the form of semantic models, we become able to provide more specific information to the dataset (such as units of measurement) that enables machines and humans to identify similar data from different sources. Since the manual creation of semantic labels and models is time-consuming, different approaches for automating semantic labeling and modeling are available. In many cases however, the semantic models must either be built completely by hand or they need at least to be revised by a human in a post-processing step (semantic refinement) due to automated approaches yielding insufficient or no result. Usually, this is done by domain experts, i.e., users who know the data very well but do have only limited knowledge of semantic technologies. Thus, supportive instruments are needed. We therefore present PLASMA, a semantic modeling tool that simplifies the (manual) component of semantic model creation needed when automated approaches cannot be used or only offer limited results. It provides an easy to use graphical user interface to lower the barrier to entry for users who lack experience in semantic modeling. PLASMA handles all interactions related to the modeling process, maintains its own ontologies and a knowledge graph and is capable of analyzing input data to identify it's schema. Moreover, the interfaces and libraries provided by PLASMA allow it to be directly integrated into dataspaces. PLASMA's modeling UI (a screencast is available on https://bit.ly/3OmeJlE) consists of the modeling area where the current semantic model is depicted as a multi-layered graph initially displaying the identified schema the user is familiar with. All graph elements can be freely arranged, e.g., to cluster nodes that represent related data. The modeler can then add semantic elements like classes, literals and relations to the semantic graph, i.e., the semantic model, using drag-and-drop interactions. Classes can be dropped on existing elements to create mappings but also onto the open modeling space to represent an unmapped class in order to provide context information. Relations between classes are created by drawing a selected relation between nodes. It is also possible to add literals to provide additional information. In summary, the operation of the PLASMA modeling UI should resemble the creation of presentation slides where users often draw nodes and connect them with arrows, providing a UI and interaction patterns familiar to users of different fields of work. In addition to the intuitive user interface, PLASMA provides supporting functionalities that further reduce the modeler's workload and speed up the modeling process. Existing models can be reused, which is especially relevant in technical environments where multiple data sources (such as sensors in machines) produce similar data sets. In addition, PLASMA provides interfaces to connect existing approaches for automated semantic labeling and modeling and to use their results in the modeling process. Finally, PLASMA's modeling interface also allows to display recommendations, thus providing the modeler with continuous options on how to improve the model. In the case of an inexperienced modeler, recommendations can give impulses on how to improve the model. Accepted recommendations, i.e., classes and/or relations, are automatically added to the model, saving modeling time. PLASMA is currently deployed in two different scenarios in which semantic models are used to annotate data. As a first application, PLASMA is used in an industrial setting to build semantic models for time series data from, e.g., different PLCs. In this scenario, PLASMA is utilized to analyze the structure of a dataset, e.g., a measurement series. Using the modeling UI, domain experts then create a semantic model for that dataset using pre-defined ontologies, as well as classes and relations defined by the experts. Once the modeling is finished, the resulting exported RDF model can be used to process the data, e.g., to integrate it into a knowledge base like an Industrial Knowledge Graph. The second application utilizes PLASMA as an integrated component inside a dataspace for smart city and mobility data. Here, data sets that are added to the dataspace require the definition of a semantic model to enhance the data quality towards the FAIR goals. Upon data extraction, the user can select which parts of the semantic model to export, using the semantic descriptions as orientation. So far, over 200 data sources have been created by municipal domain experts in the field of geo-information, who created the models without in-depth knowledge of semantic technologies.
License: CC-BY-SA 4.0 ↗
Dynamization of rainfall data - from annual series to characteristic single events as input variables for pollution forecasting - Presentation
Predicting and modeling pollution in urban water systems is crucial for effective water resource management and environmental conservation. Water pollution is influenced on one hand by the dynamics of precipitation and runoff, and on the other hand by the terrain surface structure and pollution. By dynamizing rainfall data, it is possible to infer from annual series to characteristic single events as input variables for pollution forecasting, increasing the pollution prediction accuracy.
Conventional approaches often rely on aggregated annual rainfall data, which oversimplify the complex variability and diversity of precipitation events. Consequently, these approaches yield inaccurate predictions of water pollution and hinder the implementation of targeted pollution control measures. Precipitation dynamics is a critical factor influencing urban runoff dynamics, yet it is challenging to extrapolate individual rain events or extract data for smaller localized areas. Therefore, an approach is presented here that infers characteristic individual precipitation events from long time series and attempts to capture the intricate spatial and temporal variations of rainfall in urban areas, aiming to facilitate more precise pollution forecasts in further steps.
Data management plays a crucial role in the analysis of precipitation data, as these data often comprise a combination of openly available and less accessible sources. While some of the data are publicly available, they require significant effort to be processed and prepared. Various precipitation data from different formats such as tables, rasters, and netCDFs were collected and merged for this study. The objective was to convert precipitation data into easily accessible data formats and store them in a compact manner. The collection of these data is important to create a robust dataset that provides the best possible insights into individual precipitation events and thus also enables pattern recognition in, for example, the spatial and temporal variability of such events. Here, the data were processed and transformed into the netCDF format, enabling simultaneous representation of a longer time period and spatial distribution.
Based on the collected data, individual precipitation events were characterized and conclusions were drawn about the different distribution in the urban area. Furthermore, it was examined which temporal and seasonal distribution of precipitation events plays a role in the area. Key parameters such as intensity, duration, spatial distribution, and other relevant factors are closely examined. Overall, precipitation patterns in the city should be identified, which could make the differences in urban locations visible and highlight characteristic features.
In subsequent steps, these findings can be utilized as inputs for pollution forecasting in urban regions. The urban environment includes diverse surface textures, such as impervious materials like concrete and asphalt, as well as vegetated areas and open spaces, which collectively influence the quality of precipitation and subsequent water discharge. These surfaces can have distinct effects on the quality of precipitation as it interacts with the urban environment. For example, impervious surfaces can lead to increased runoff and contribute to the wash-off of pollutants accumulated on the surfaces, resulting in degraded water quality. Understanding how the dynamics of precipitation events play out in an urban area can help to better draw conclusions about the water pollution levels.
In subsequent stage, the characterization of individual precipitation events also aims to establish connections with measured groundwater levels. This linkage facilitates the identification of infiltration parameters through soil moisture analysis. Such an approach enhances the understanding of the impact of individual events on groundwater levels and enables a more comprehensive analysis of the relationships among rainfall, soil conditions, and groundwater dynamics using soil moisture data.
Ultimately, optimizing water resource management in an urban area can be carried out by using the dynamics of precipitation and the distinctive attributes of characteristic rain events for pollution forecasts. The insights gained can push the development of robust strategies for climate change adaptation and sustainable urban water management, safeguarding water resources and promoting long-term environmental sustainability in an urban area.
License: CC-BY-SA 4.0 ↗
Raising Awareness for Data FAIRness. An Approach to Developing FAIR Data Literacy in Engineering Sciences Undergraduate Courses - Presentation
The FAIR Data Principles[1] are an established guideline for research data management (RDM) and data sharing across academic disciplines. While recognizing that access to some research data must be restricted for legal or contractual reasons, the FAIR Principles define a set of conditions that facilitate the reusability of research data in scientific and commercial contexts. To enable such re-use of data by both humans and computers, good quality metadata and documentation are key.
Raising awareness of the FAIR principles and building expertise in managing data in a FAIR-compliant manner should start early in the academic career of researchers. These are critical skills and an important contribution to data literacy. However, imparting such skills among undergraduate audiences can be challenging because they often have little or no experience with data management and may struggle to identify reliable and well-curated data sources, especially in online contexts.
This talk models the FAIR data principles into teaching scenarios as a contribution to data literacy for undergraduate students. An ideal vehicle are database training sessions offered by course instructors or library staff to undergraduates because such courses have long been helping students develop information literacy.[2] This skill of critically reflecting on information-seeking behavior and the use of information to create new knowledge can be expanded and adapted to introduce students to FAIR-compliant research data management. By working with databases in a supervised environment, students learn to interact with structured collections of data and to manage and process their results for later reuse. The FAIR Principles are particularly useful for distinguishing well-curated and standards-compliant data collections from websites without a clear curation policy.
The proposed model entails a low-threshold set of five criteria against which students check databases. These five criteria do not replace the FAIR principles, but they steer students towards a critical engagement with the databases and web resources they use in their everyday studies. In this way, students are encouraged to think about best practices for managing and sharing research data, which will inform their future approach. It will also lay the foundations for the more advanced form of information data literacy.[3]
This teaching model was developed by subject librarians and RDM support librarians at Bern University Library (Switzerland).[4] It was successfully used in courses for undergraduate students of German literature, who mainly work with textual data. This talk shows how to transfer the model to an engineering sciences context. The target audience are RDM support staff, library staff, and undergraduate instructors who wish to integrate the FAIR data principles into their courses. This talk will (1) contribute to the discussion of adapting the FAIR principles to undergraduate teaching scenarios, especially in engineering sciences, and (2) provide a reusable model that can be implemented in concrete course settings.
[1] “FAIR Principles,” GO FAIR. https://www.go-fair.org/fair-principles/ (accessed Jun. 30, 2023).
[2] American Library Association, “Framework for Information Literacy for Higher Education,” Association of College & Research Libraries (ACRL), Feb. 09, 2015. https://www.ala.org/acrl/standards/ilframework (accessed May 09, 2023).
[3] J. Carlson and L. R. Johnston, Eds., Data Information Literacy: Librarians, Data, and the Education of a New Generation of Researchers. Purdue University Press, 2015. Accessed: May 13, 2022. [Online]. Available: http://doi.org/10.26530/oapen_626975
[4] G. Schreier and U. Loosli, “Data Literacy. Eine Einführung für Geistes- und Kulturwissenschaftler*innen,” Jun. 13, 2023. Accessed: Jun. 30, 2023. [Online]. Available: https://doi.org/10.48350/183384
License: CC-BY-SA 4.0 ↗
Geodata Infrastructure for the Management of Research Data in Railway Domain - Presentation
Railway transportation is being used by millions of people every day for travel as well as transportation of goods and so, its continuous operation is essential. A database containing up-to-date information on railway infrastructure and its condition is an important asset to maintain railway infrastructure, as well as to develop new technologies and algorithms for managing the data collected from the infrastructure and monitoring its condition [1, 2]. The Institute of Transportation Systems (DLR-TS) of the German Aerospace Center (DLR) carries out research in the area of monitoring the condition of railway infrastructure through the use of data measured by sensors mounted on in-service trains. Massive amounts of measurement data are collected from various sensors mounted on railway vehicles that operate on a regular basis [2, 3, 4]. These datasets are georeferenced, fused with other openly available datasets, such as OpenStreetMap (OSM) and weather data from Deutsche Wetterdienst (DWD) and analyzed by multiple algorithms, in order to detect anomalies and potential problems with railway infrastructure. Researches at DLR-TS need the raw and the processed datasets to be persisted in a single platform within the DLR. This platform must be capable of making the data available to DLR-TS researchers, project partners and other stakeholders over a long period of time for analysis and visualization. Thus, there is a demand for sustainable and scalable management of data within DLR-TS.
To address these demands, DLR-TS is currently developing a platform known as “Transportation Infrastructure Data Platform” (TRIDAP) as a part of the DLR-funded cross-domain project called “Digitaler Altas 2.0” [5]. This presentation explains the scope and features of TRIDAP, its current status and the planned development activities.
TRIDAP aims to support holistic management of traffic infrastructure data collected from both road and railway domains. The platform supports the storage, analysis and sharing of georeferenced as well as non-georeferenced datasets. Storage of digital railway infrastructure maps such as, railway networks, position of signals, level crossings, balises and switches is also supported. The condition of the infrastructure, for example, status of signals, status of level crossings and level of degradation of rails, can also be stored in TRIDAP. For condition monitoring applications, it is also interesting to have information on changes in the railway infrastructure, (such as construction of new infrastructure or removal of existing infrastructure) and management activities (such as repair and improvement of existing infrastructure) carried out in the past, which can also be stored in the platform. In order to make these datasets Findable, Accessible, Interoperable and Reusable (FAIR), the system also enables the storage of sufficient metadata as well as supports the publication of datasets through the use of open-source software GeoServer and GeoNetwork. Furthermore, DLR-TS has developed a HDF5 data model for the storage of dynamic data collected from various sensors mounted on railway vehicles [2]. Most of the static and dynamic data are georeferenced and are stored in a common space and time reference frame – World Geodetic System / WGS84 and Coordinated Universal Time (UTC).
The platform contains instances of various big data open-source software, such as Apache Kafka, Apache Flink, Apache Spark, to process and analyze the data stored in the system through the development of stream and batch processing applications. The results of data analyses are written back into the system to keep raw and processed datasets together. To carry out fusion of measurement and weather datasets, we are currently developing a python-based tool to download data from Deutscher Wetterdienst (DWD) for a user-defined region and time period directly into the data processing application. Other sources of weather data are planned to be integrated in the future. In order to provide a high-quality service to the researchers, it is inevitable to ensure high availability and optimal performance of the platform. To achieve this, we are integrating all components of TRIDAP into a monitoring framework that uses a monitoring tool called Prometheus and a visualization tool called Grafana.
Furthermore, we are developing another python-based tool to validate the data being stored in the system. For this purpose, we define a set of validation rules together with the team of researchers who are responsible for setting up the sensors, collecting and using the sensor data. The first module in the validation tool deals with dynamic live data we receive from railway vehicles in the field. When validation errors are identified, the team who set up the sensors are immediately informed, so that they can resolve the issue as quickly as possible.
The geo-datasets stored in TRIDAP are shared with stakeholders in standardized data formats through the use of GeoServers. GeoNetwork is being used to setup a geodata catalog that enables easy search and access to datasets stored in the platform. The GeoNetwork uses metadata standards such as Dublin Core and ISO/TS 19139 to document metadata. It is also planned to connect GeoNetwork with the research data repository (FDR) of the DLR to obtain a persistent ID (PID) for the datasets on-demand. Certain datasets stored in the platform are confidential and have restricted access. This is currently being implemented through the definition of multiple users, roles and data security rules in the GeoServer as well as the data storage layers. However, we intend to switch to the use of Keycloak in the near future to handle restricted access to data. Keycloak is a user federation tool that provides strong authentication and fine-grained authorization.
References
1. Shankar, S.; Roth, M.; Schubert, L.A.; Verstegen, J.A., “Automatic Mapping of Center Line of Railway Tracks using Global Navigation Satellite System, Inertial Measurement Unit and Laser Scanner”. Remote Sens., 2020, doi: https://doi.org/10.3390/rs12030411
2. Shankar, S.; Heusel, J.; Böttcher, O.; und Patil, A.J.; Baasch, B., „Management von großen Sensordatenmengen für die Digitalisierung und Automatisierung im Bahnbe-reich“. ETR - Eisenbahntechnische Rundschau (12), DVV Media Group, 2022, pp. 45-49, doi: https://elib.dlr.de/188041/
3. Heusel, J.; Baasch, B.; Riedler, W.; und Roth, M.H.; Shankar, S.; Groos, J.C., “Detect-ing corrugation defects in harbour railway networks using axle-box acceleration data.” Insight (64), The British Institute of Non-Destructive Testing, 2022, pp. 404-410, doi: https://doi.org/10.1784/insi.2022.64.7.404
4. Baasch, B.; Heusel, J.; Roth, M.H., Neumann, T., “Train Wheel Condition Monitoring via Cepstral Analysis of Axle Box Accelerations”, Applied Sciences, 11 (4), Multidisci-plinary Digital Publishing Institute (MDPI), 2021, doi: https://doi.org/10.3390/app11041432
5. Digitaler Atlas 2.0 – domänenübergreifende Softwareanwendungen und Geodateninf-rastrukturen für die Prozessierung von Geodaten, URL: https://verkehrsforschung.dlr.de/de/projekte/digitaler-atlas-20-domaenenuebergreifende-softwareanwendungen-und-geodateninfrastrukturen, Last accessed on 30.06.2023.
License: CC-BY-SA 4.0 ↗
Just a word with you: How vocabularies and ontologies increase the findability and reusability of your research. A workshop report. - Presentation
Everyone who has ever published a research paper knows this: keywords need to be added to the bibliographic information to describe the content as briefly and precisely as possible. What may seem rather annoying to some is actually the entry into an interesting field of possibilities to make your research more findable. The assignment of keywords or even the use of certain words or phrases in the title or abstract helps to make the content easier to find and more visible. A basis for this is provided by the use of controlled vocabularies, i.e. a catalog of predefined terms. Such vocabularies are already used in numerous scientific information systems, such as literature databases like the online catalog of the FID move (www.fid-move.de/en/online-catalogue/) or the Mobility Compass (www.mobility-compas.eu). Here, they enable linking all kinds of entities with each other (e.g. publications, data sets) to form a networked structure and thus providing better findability of resources and supporting the users’ information retrieval.
But this is only the tip of the iceberg. The easier it is to interpret the contents of publications in the context of various applications, the better they can be linked to other results and thus increase their reusability. Particularly in the area of research data, there is very high potential here for the entire cycle from data collection to data publication. Accordingly, there are already numerous proposals or standards for describing individual research data. However, since these often originate institutionally and are in part not easily machine-readable, data silos still form or the effort required to link different research data is still high. In the context of NFDI4Ing, ontologies such as ListDB Onto (www.gitlab.com/listdb/onto) are therefore being developed and made available in close collaboration with researchers. The aim of those ontologies is supporting researchers in making their data available and reusing it. For example, ListDB Onto enables the networked description of video data, associated processes, people involved or tools used. It thus forms an important building block for interoperability between different video-related metadata.
The session is a workshop report, which summarizes the several years of experience of the presenters in the creation and integration of controlled vocabularies and ontologies. On the one hand, the added values and usage scenarios for science will be discussed. On the other hand, challenges and lessons learned in building vocabularies will be elaborated. Thus, the talk addresses researchers as well as employees in scientific infrastructures.
License: CC-BY-SA 4.0 ↗
A Digital Twin Framework for Field Data and Distributed Systems - Presentation
Digital Twins (DTs) are state-of-the-art technologies that have been widely exploited in recent years to facilitate various processes, such as manufacturing, healthcare, transportation, simulation, and accurate forecasting. They represent a virtual replica of a physical entity or state and can be seen as a major element in Industry 4.0 showing high potential for tackling different challenges in Research Data Management (RDM). Despite the considerable benefits of DTs in industry and engineering, the existing DTs are primarily domain-dependent and do not generalize to more intricate cases, especially when the physical twin corresponds to a field-dependent entity within distributed systems. This then hinders a straightforward implementation of DTs by researchers.
In this contribution, we therefore ask how the concepts of DTs can be generalized to the distributed systems and to the more intricate physical entities that encompass field and field-related data. We propose a generalized DT framework for field data and distributed systems and as a first step, define the required components and describe their specific characteristics in connection to the investigated physical entity. The functionality of such DT components will be then specified and detailed. In this regard, we make use of previous models and define novel interaction between the different components of the physical and virtual spaces.
Our investigation focuses on field data of distributed systems and aims to establish an effective data flow between the different components of our DT. This task is particularly challenging since the investigated distributed systems contain different moving and non-moving physical entities and thus the exact definition of the DT components becomes intractable. To address this, we consider three components in line with our research objectives: (A) a physical space which encompasses physical entities that will be modeled and analyzed, (B) a virtual space containing DTs that replicate the considered physical entities and provides the basis for the analysis and investigations, and (C) communication which links the physical and virtual spaces. The latter plays a vital role in DT technologies which distinguishes them from other simple simulation techniques. Here, communication refers to the exchange of data and information between the two spaces forming an end-to-end model given the proposed framework.
Within the virtual space, we define various digital shadows, each investigating a specific aspect of the considered physical entity. Additionally, we introduce a digital master that stores metadata and requirements of digital shadows. The prototype will be constructed in digital master which instantiates the phases and processes of digital shadows. Then, as our main contribution, we describe how dataflow (as a form of communication) between these different spaces and entities can be established. We subsequently investigate four different types of communication:
1. Physical-to-physical communication: In the considered distributed systems, multiple physical entities (e.g., devices, field and environment, human, etc.) are interconnected which makes the investigations or operations more challenging. We define the relations and services between these entities using a physical-to-physical communication.
2. Physical-to-virtual communication: This corresponds to the transfer of data from the physical space to the virtual space. We here consider field data collected in the physical space which should be further processed and analysed in the virtual space using the proposed DT framework.
3. Virtual-to-virtual communication: The considered DT components are in fact connected with each other to form a network for information and output exchange. As mentioned above, we here propose a digital master in combination with different digital shadows that each is responsible for certain tasks. The interoperation between these different components will be then defined using a virtual-to-virtual communication. Besides, we here discuss how different DTs can be combined to establish an aggregated system (referred to as aggregated DTs).
4. Virtual-to-physical communication: Finally, the output will be transferred from the virtual space to the physical space. This final step, referred to as virtual-to-physical communication further completes the end-to-end framework.
The communications above ensure an active data and information flow throughout the proposed DT framework. We consider a case-study developed in archetype Golo of the NFDI4Ing consortium, in which the description of the corresponding distributed system is based on three different physical entities: a vehicle equipped with required tools and sensors; public road and traffic, and also the driver of the vehicle. We describe each of these entities and theoretically discuss how the investigated DT framework can be used.
License: CC-BY-SA 4.0 ↗
Community-based RDM training portfolio for the engineering sciences - Presentation
With research becoming more data-driven, the sustainable handling of research data has gained more importance during the last years. Funding agencies are increasingly demanding a dedicated Research Data Management (RDM) in research projects. However, next to the additional effort, researchers need the respective skills and competencies to handle and manage data collected and used in their research.
In the course of this development, the RDM community has already conducted various trainings and created several training materials. Especially self-paced format such as Open Educational Resources (OER) and Massive Open Online Courses (MOOCs) are chosen due to best scalability and temporal and spatial independence in learning. The covered training topics are typically rather generic to be applicable for a large group of researchers. Nevertheless, there is a need for discipline-specific trainings to gain increased acceptance in the respective subject-specific community and to address particular aspects and challenges of them. In NFDI4Ing, the National Research Data Infrastructure for the Engineering Sciences, this need has been raised by the engineering community.
Such demand is comprehensible but implies challenges in conception and realisation: Typically, communities from one large field are rarely homogeneous. For the engineering sciences, this ranges from production technology to material sciences to civil engineering. Therefore, it requires adjusting the generic training contents to the respective subject accordingly. A second (general) challenge is to consider is the diverse experience and competency levels as well as learning objectives for each target groups (like bachelor, master, PhD student, data steward etc.).
In NFDI4Ing the Base Service S-6 together with the Community Cluster CC-2 are responsible for preparing trainings for basic RDM topics specifically designed for the engineering sciences. Learning objectives have been defined according to the competency levels (rf. matrix in https://doi.org/10.5281/zenodo.7034477). In their process of creating learning materials, the S-6/CC-2 team uses the following methodical approach: First, existing (generic) RDM trainings have been identified. These are presented in an overview list and are reused – according to the respective license – as foundation for the preparation of engineering-specific trainings. Next to the training contents itself, various training formats (like self-paced trainings, workshops, train-the-trainer etc.) and target groups have been identified and defined. This has been the starting point for the creation and publication of first (engineering-specific) self-paced RDM trainings in NFDI4Ing’s Education Repository [https://education.nfdi4ing.de].
Since the NFDI4Ing S-6/CC-2 team cannot cover all engineering research disciplines in detail by their own, a community-based approach is chosen: The created self-paced trainings are discussed with everyone interested in a so called “Special Interested Group” (SIG) for “Basic RDM training”. For each of these meetings with the community a topic is chosen and the respective existing training is presented. Based on this, participants are asked for feedback and suggestions. This aims to develop RDM trainings tailored to the needs of the engineering science’s community and to enrich (previously rather generic) trainings with an engineering perspective. In terms of the engineering-specific orientation, the community is asked for examples or anecdotes to illustrate the content practically. Even if it is not expected that such examples are applicable for everyone in the heterogeneous engineering community, they represent the community’s individual needs and appear helpful to personally identify with the topics. Integrating negative examples – sometimes referred to as “data horror stories” – into the trainings is a way to share experiences and to highlight potential consequences when (not) following the recommended training contents. Representation formats for all these examples can range from short texts to audio statements, animations, or videos integrated in the web-based trainings. Beyond this, a special focus is currently on adding interactive elements to the trainings. Quizzes, assignment tasks and check questions are also used as diversified learning control and to reinforce the taught contents. Technically these are H5P elements, a format for various interactive elements, which can be exported for websites.
In summary, the community-based approach enables to provide basic RDM trainings specific for the community of the engineering sciences and to align on the community’s needs. The trainings are incrementally and continuously updated.
Next to the engineering community, the S-6/CC-2 team is networking with other training initiatives to make the trainings publicly known and to exchange experiences, like in the cross-consortia’s NFDI Section “Education & Training”. With platforms like DALIA (Data Literacy Alliance) as well as OERSI (Open Educational Resources Search Index) an enhanced dissemination of the trainings is intended.
In this conference talk we present our service offerings as well as our approach how the integrated the community from the engineering sciences into the development of discipline specific RDM trainings. The presentation will conclude with a demonstration of our training repository and an outlook to the future next steps. Everyone from the engineering community as well as from infrastructure services (like IT centres and libraries) is invited to contribute on a subject, generic or didactical level.
Acknowledgement
"The authors would like to thank the Federal Government and the Heads of Government of the Länder, as well as the Joint Science Conference (GWK), for their funding and support within the framework of the NFDI4Ing consortium. Funded by the German Research Foundation (DFG) - project number 442146713."
Authors
Marcos Galdino, Canan Hastik, David Hecker, Christian Langenbach, Mario Moser, Janna Neumann, Ute Trautwein-Bruns, Kerstin Wedlich-Zachodin
License: CC-BY-SA 4.0 ↗
Workflow elements for the creation of rich metadata in collaborative research environments - Presentation
High-quality metadata production and management are vital for interoperability, sharing, and reuse of research data. Metadata enables data discovery, reproducibility, and effective data management. This presentation introduces building blocks for the design of workflows aimed at creating rich metadata in collaborative research environments.
Our work addresses two key necessities:
Firstly, within the larger research data management (RDM) community, there is a growing demand for standardized and well-documented metadata, as highlighted by the FAIR principles and the concept of "rich" metadata. However, the precise definition and scope of what constitutes "rich" metadata remain ambiguous. In order to contribute to a comprehensive understanding of "rich" metadata, this presentation proposes a set of metadata categories that analyze the metadata creation workflow throughout the data lifecycle.
Secondly, complex research projects with extensive amounts of data require division of labor and coordination among various domain experts. As the volume of data and the need for rich metadata increase, dedicated data management roles and robust data management practices become crucial. To facilitate clear communication about data management among different stakeholders in the research process, an expressive language is required. This presentation makes a contribution in this direction by focusing on the creation of metadata throughout the data lifecycle.
Typically, metadata is defined as "data about data," which represents an object-centric view. However, when describing workflows, it is critical to consider processes as well.
Therefore, and firstly, a workflow-oriented analysis of metadata must acknowledge that data is always the output of a particular procedure. Both the procedure and its output generate metadata. This notion is already established within the RDM community, evident e.g. through the "processing step" class in the "Metadata4Ing" (M4I) ontology. High-level examples of processes are the stages of the data lifecyle, i.e. planning, collection, analysis, etc. Each of them comprises additional mid- and low-level processes, which create intermediate or auxiliary outputs.
Secondly, before data is created or transformed, both the procedure and its output are usually planned and specified, resulting in metadata that is not extracted from the data but injected into it. Examples for injected metadata are the address of a software repository (specifying a process), or a database schema (specifying an output). Extracted metadata are e.g. performance metrics (of a process) or a file checksum (of an output).
Thirdly, metadata itself is also data, as noted by many sources. Consequently, yet rarely recognized in discussions, metadata creation is recursive. By acknowledging the existence of meta-metadata or second-order metadata, the RDM community has an opportunity to advance the standardization and harmonization of primary metadata. For example, the utility of a data description (primary metadata) may be improved by an explicit and formal format specification (meta-metadata), facilitating e.g. machine processing.
Fourthly, beside the one just given, there is an additional workflow-related interpretation of the idea that "metadata is data": A data point may be enlisted as metadata for another data point, even though there is no immediate dependency between the two, and both make sense on their own. As an example, consider a time series of force measurements (data) at the joints of a robot traversing a plane, and the incline of that plane (independent metadata). This is in contrast to dependent metadata, like e.g. the measurement unit of the force values.
These four workflow-related metadata dimensions (procedure vs. output, injected vs. extracted, dependent vs. independent, recursion) are orthogonal to established categories that primarily consider the purpose or semantics of metadata, such as structural, descriptive, or administrative classifications.
To demonstrate the practical implications of our concept, we present examples of workflow-oriented metadata categories in two ongoing applied projects: DeeperSense and RoBivaL. These projects are being conducted by DFKI in close relation with the NFDI4Ing TA Golo. Additionally, we illustrate how these building blocks can be assembled to connect all stages of the data lifecycle in real-world research scenarios. Specifically, we discuss the compatibility of our processing metadata examples with the M4I processing step class, and explore the value of NFDI4Ing services at different stages of the research process.
We also address potential challenges and risks associated with metadata generation and management. The presentation highlights the risk of scope explosion, where metadata generation might be conflated with general knowledge management. We emphasize the importance of distinguishing between metadata management and knowledge management while acknowledging the potential synergies and lessons that can be learned from existing knowledge management approaches.
In conclusion, this presentation showcases a comprehensive framework for analyzing and generating rich metadata in collaborative research environments. By categorizing metadata along multiple dimensions and recognizing the recursive nature of metadata generation, the framework aims to provide practical guidance to researchers, data managers, and stakeholders on effectively managing metadata throughout the data lifecycle. The proposed framework, supported by case studies, contributes to the advancement of FAIR principles and promotes the adoption of standardized and high-quality metadata practices in research communities.
License: CC-BY-SA 4.0 ↗
N2O emissions forecasting with neural networks - expanding the training database using deterministic models - Presentation
Background
Due to its high global warming potential of 265 CO2 equivalents, even minor emissions of the greenhouse gas nitrous oxide (N2O) can have a significant impact on CO2e balances. N2O emissions can also occur at wastewater treatment plants. During biological nitrogen elimination, N2O can be formed as a by-product especially when unfavorable operation conditions are prevailing (e.g. oxygen deficiency, overloading, inhibition effects…). The occurrence of N2O emissions normally underlies high temporal and spatial variations. N2O emissions can be minimized by an adapted mode of operation. Various control strategies have already been developed for this purpose; however, there are only a few large-scale implementations to date.
Currently, most of the control strategies are based on direct processing of online measured parameters. However, these measurements cannot realistically represent the actual spatial and temporal variation of operating conditions in the reactor, especially since not all relevant parameters can directly be measured. Furthermore, the installation and maintenance of the measurement technology is quite complex and expensive. For advanced control tasks such as minimizing N2O emissions, the additional use of calibrated digital models will therefore gain in importance ("digital twin").
Deterministic models based on biological and physical process equations are already applied to simulate the mechanisms of N2O formation and emission. These models can be calibrated using data from individual N2O measurement campaigns. A disadvantage is, however, that only the (known) implemented processes have an influence on the result; other (unknown) effects cannot be represented.
Approach for combining deterministic models and neural networks
To expand the possibilities of modeling support, the use of neural networks for emission forecasting is currently being discussed. These networks do not depend on explicitly defined process equations; instead they independently construct a model during the training phase.
For the training of neural networks an extensive database is needed, that directly or indirectly includes all parameters significantly influencing the network output. The training data sets must have sufficient variation to be able to represent different operating situations or operating faults with high accuracy.
In practice, however, exactly these high-quality data are not available. Especially for operational disturbances, there is often very little data available, since these situations usually have occurred only occasionally. Thus, there is an urgent need to close this data gap. Therefore, the existing measurement data is used to calibrate a deterministic model. Subsequently, the model is employed to expand the training data sets by calculating resulting N2O emissions and other operational parameters for varying input data. The data sets generated in this way are then used for the neural network training.
Through this innovative approach the knowledge stored in the process equations of the deterministic model can be effectively utilized. Furthermore, the neural network takes additional unknown/ unconsidered effects into account after training is completed. Another advantage of the presented approach is the short computation time of the trained network, which strongly supports proactive automatic optimization of the treatment process.
A major challenge is still the evaluation of the reliability of the network output. The output data depends on the quality of the trained network (and therefore on the quality of the deterministic model) and also on the distribution of the specific network inputs. So, the network can only provide reliable results if the inputs all lie within a range covered by the training data. Accordingly, the predicted N2O emission must always be specified together with an error probability.
Application of the combined approach
The approach presented here was subject to a first application using data from pilot plant operation. A deterministic model was established using the operating data and the results of N2O measurements. With the aid of the model, the training data sets (influent data + operational settings + resulting N2O emission) were generated/ expanded. In a second step, the data sets were employed to train the neural network that is foreseen to predict N2O emissions for defined operating situations. Finally, the neural network was applied to determine the optimal operating settings for the pilot plant, which resulted successfully in a reduction of emissions.
Conclusions
The use of deterministic models to generate input data for neural network training purposes can compensate essential weak points of both modeling approaches. This approach has the potential to establish a very valuable tool for N2O emission minimization on wastewater treatment plants. However, an evaluation of the input data is required before applying the network to ensure that the result is reliable (inputs must be in the trained data range).
License: CC-BY-SA 4.0 ↗
Experience with benchmark datasets in the heterogeneous data landscape of photogrammetry and remote sensing - Presentation
The field of photogrammetry and remote sensing has a wide range of applications. Our research field deals with the whole data life cycle from data acquisition with different sensors to data processing and reuse of data for methodological developments. Furthermore, the data products of photogrammetry and remote sensing often serve as the data basis for other disciplines. For example, we derive digital terrain models form airborne laser scanning data and those resulting models are subsequently used by geoscientists as input data for their research. As a result, the research data and products exist in different processing levels. Thus, it is even more important to publish and manage research data in an understandable manner.
However, application of research data management in the associated community, the international society for photogrammetry and remote sensing (ISPRS), is challenging. Specific best practices for the publication of research data do not yet exist. In addition, the heterogeneous research data can be assigned to different applications, making it difficult to assign them to a single NFDI consortium. Thus, services of NFDI4Ing, NFDI4earth and NFDI4DataScience are considered. While some helpful tools for research data management are already available at NFDI4Ing, NFDI4earth and NFDI4DataScience are at the beginning. However, it should be noted that in the direction of geoscience and thus in the NFDI4earth domain, subject-specific data repositories have been available for some time, which show overlaps with the ISPRS community. In contrast, with the open research knowledge graph (https://orkg.org/), which used among others Papers With Code (https://paperswithcode.com/), the NFDI4DataScience provides a strong connection between paper and benchmark datasets.
In order to improve the dissemination of research data management in the ISPRS community, especially the publication and reuse of benchmark datasets, the BeMeDa and BenchPub projects were created. The former project primarily involves uniformly recording metadata for existing benchmark datasets, resulting in an enhanced search feature within its dedicated demo platform (https://benchmedata.org/) [1]. This also allows sorting of the datasets. The current BenchPub project, in contrast, is concerned with the development of a best practices guideline for the publication of datasets, which is specialized in heterogeneous data in photogrammetry and remote sensing and enables a sustainable usage of the data. The key components of both projects are the use and development of a metadata concept and the consideration of a domain specification and standardization.
Due to the increased amount of published papers, it is difficult to identify research gaps and associated data. Thus, a keyword analysis is used to specify the photogrammetry and remote sensing domain. Due to spelling variations, the keywords are grouped by their similarity. This results in the top four keywords: deep learning, point cloud, classification and lidar. According to this keyword analysis, 3D data has great importance. This means that it must be possible to manage 3D data in data repositories in addition to 2D image data. For this, we compare different data repositories, general and subject-specific. We establish evaluation criteria that encompass factors such as the maximum data upload capacity, associated costs, adherence to standardized metadata schemas, and adherence to the FAIR principle.
[1] Budde, L. E., Schmidt, J., Javanmard-Ghareshiran, A., Hunger, S., and Iwaszczuk, D.: Development of a Database for Benchmark Datasets in Photogrammetry and Remote Sensing, ISPRS Ann. Photogramm. Remote Sens. Spatial Inf. Sci., V-1-2022, 187–193, https://doi.org/10.5194/isprs-annals-V-1-2022-187-2022, 2022.
License: CC-BY-SA 4.0 ↗
Chemical Process Data for Research - Presentation
The chemical industry, one of the world’s most important branches of manufacturing, will be fundamentally changed by data-driven methods from Artificial Intelligence (AI) – both the way processes are designed and the way processes are operated will be deeply affected. Hopes and expectations in chemical companies are high, but so are the hurdles. The data from chemical processes differs fundamentally from the data in areas in which methods from AI have excelled so far: chemical process data are sparse (often related to basically only a narrow operation window of a plant), highly correlated, heterogeneous, and subject to uncertainties. Furthermore, erroneous predictions may have disastrous consequences in chemical processes, so that the demands on the quality of the results from AI methods are extreme: they must be reliable, explainable, interpretable, and trustworthy. Important progress in research on AI methods in chemical process engineering will be needed before fruits from AI can be harvested in the chemical industry. A key issue here will be to incorporate the important body of physical knowledge on the chemical processes into the AI methods, thus creating hybrid methods.
However, there is a major obstacle to academic research on AI methods for chemical processes: practically no chemical process data is presently publicly available. For obvious reasons, companies are reluctant to share the data on their processes. And even if they would, providing such data in ways that would be useful for research would pose substantial challenges. In particular, an ontology providing a frame for this endeavor is still lacking.
An alternative to using industrial data on chemical processes is to produce suitable data for research outside the industry. This approach is taken in the DFG Research Unit FOR 5359 “Deep Learning on Sparse Chemical Process Data”, which was established in 2022. Two processes are operated by FOR 5359: a continuous pilot plant for producing synthetic fuels at TU Munich and a batch-distillation plant equipped with conventional and non-conventional sensors at RPTU Kaiserslautern. The new data is used in FOR 5359 to develop methods for deep anomaly detection in chemical processes. This will require the development of suitable data formats based on a corresponding ontology of chemical process data. The experimental data will be augmented by simulation data as well as by synthetic data generated by AI algorithms trained on experimental and simulation data. This is necessary as, despite all efforts, the amount of experimental data that can be generated is small compared to what is typically required for training AI methods. The new data will also be published and can be used freely for academic research by any interested party.
Specific questions to be addressed thereby include:
• The relation of experimental data and simulation data, including questions related to the required post processing to create suitable hybrid data sets.
• The relation of conventional data, as it can be used directly for comparison with process simulation data (e.g., data on temperature, pressure, composition, flows) to non-conventional data (e.g., video, audio, spatially resolved data, meta-data).
• The relation between process data and related data, such as data on thermodynamic properties.
• The challenges related to the use of synthetic data generated by AI algorithms trained on experimental and simulation data, including the question, if this can meaningfully augment the data base.
In the presentation, we will first describe the challenges related to chemical process data for AI applications and compare the situation to other fields, where AI is already successful. We will then introduce the approach of FOR 5359, and discuss the questions listed above. Finally, we will present first results from deep anomaly detection on chemical process data. The data basis for this was the only publicly available data on a chemical process, the Tennessee Eastman Process, which is, however, only simulation data. Nevertheless, it was used here for a systematic evaluation of about 30 different anomaly detection methods from the literature, demonstrating principal feasibility of the approach.
License: CC-BY-SA 4.0 ↗
Datacubes for Spatio-Temporal Engineering - Presentation
In science and engineering “fields” as defined in physics are common: space-time varying phenomena which get discretized in some way when sampled through sensors or generated as simulation output, for example viscous flow in CFD, stress and temperature flow in solid materials, satellite image timeseries in Earth Observation, and weather forecasts, to name but a few. Very often the resulting data structure in a computer is that of a regular or irregular grid, due to the size of the phenomenon typically distributed over a possibly larger number of files. This sensor-centric file set classically is served to users or further processing units. However, this low level of semantics frequently makes handling cumbersome, requires unnecessary deep knowledge of technicalities, and may be inefficient.
Another way of offering data is user-centric, rather than sensor-centric, through an approach where one phenomenon appears as one object, in case of gridded data so-called datacubes. Such datacubes can be multi-dimensional – such as 1D sensor timeseries, 2D images, 3D x/y/t image timeseries or x/y/z volumetric data, 4D x/y/z/t flows, etc. – and the grid structure can be regular or irregular. On this semantic model services can be built with rich, semantically adequate functionality. This starts with geometric extraction through “bounding boxes” to trim or slice a cube and extends over polygon/polytope clipping into analytics and ML. Generally speaking, as the mathematical structure of a datacube is that of a tensor, the corresponding service functionality is based on Tensor Algebra.
The rasdaman (“raster data manager”) datacube engine follows a database approach supporting management and analytics of large gridded data as n-D arrays. Internally, large arrays get partitioned into tiles whereby the tiling strategy is freely configurable by the administrator. To the clients this internal structure remains hidden, it is just a tuning factor. Access is through a dedicated array query language, rasql, which offers operators streamlined for arrays. Meantime, this language has been added to the SQL standard. Rasdaman instances can be federated allowing creation of a single datacube pool from autonomous deployments, including distributed datacube fusion. Access control is possible down to the granularity of single array cells.
Applications done with rasdaman include many domains, such as CFD, human brain research, genetics, cosmology, and Earth sciences. In particular in the latter domain rasdaman is in active use, such as on satellite data, atmospheric data, radio networks planning, etc. The EarthServer federation running rasdaman currently incorporates 160 Petabytes with nodes from Europe to Taiwan.
In our talk we present the rasdaman design, architecture, applications, and standardization impact and underpin it with several live demos.
License: CC-BY-SA 4.0 ↗
The Workbench of the FID BAUdigital - A Platform for Research Data Management in Civil Engineering, Architecture and Urbanism - Tool demonstration
The FID BAUdigital aims to provide specially tailored services for researchers in the fields of civil engineering, architecture, and urban studies, with a focus on digital methods and technologies. This paper introduces the Workbench, a platform developed by the University and State Library Darmstadt (ULB) within the domain-specific repository infrastructure of the Fachinformationsdienst (FID) BAUdigital, designed to facilitate the provision and reuse of research data guided by the principles of Open Access and FAIR utilizing services provided by NFDI4Ing base services of measure S-3. The Workbench aims to expand the corpus of available born-digital and retro-digitized data for research in these fields through data acquisition, metadata curation, and harvesting, while actively involving the research community through case studies.
The Workbench provides a user-friendly interface for publishing research data, allowing data to be uploaded, metadata to be enriched semi-automatically, additional metadata to be entered via forms, and 2D and 3D previews to be generated automatically. Technically, the Workbench utilizes S3 storage for data, a triple store for metadata, and a modular, container-based infrastructure based on Kubernetes. When data is uploaded, various processing tasks are triggered, including data conversion, preview creation, and metadata extraction as key functions of the Workbench. As example, 3D data is converted to the open GLTF format using a Blender instance and transformed into web-ready 3D models with the help of Rapid-Compact. Blender is also used to render 2D views that can be displayed along with the reduced 3D models in the Workbench graphical user interface. Furthermore, metadata extraction from domain-specific formats such as IFC is automated, providing relevant information about the represented buildings, such as the number of floors.
The Workbench aims for demand-oriented development, with the goal of being accepted by a large number of users. Monolithic metadata solutions are not suitable due to the breadth of disciplines and the associated heterogeneity, complexity, and methodological dependencies of the data. The NFDI4Ing base services of measure S-3: metadata & terminology allow for the collaborative development of modular metadata schemas with researchers, making them reusable and adaptable. Therefore, the technical infrastructure of the Workbench is designed to utilize these services, especially the Metadata Profile Service (S-3-1) for the creation and storage of profiles based on vocabularies from the Terminology Service (S-3-2). These profiles can then be retrieved by the Workbench and used for the ingestion of research data. Initial application profiles are currently being exemplified with a variety of test data from the research community and photogrammetrically 3D-scanned architectural models within the scope of the FID BAUdigital. Furthermore, within the NFDI4Ing Seed Funds project "RDM-Workflows for construction engineering and architecture," additional application profiles for buildings, stakeholders, and drawings are being developed, as well as a workflow and documentation for the whole process.
For users, the Workbench serves as an easy-to-use search interface for research data, as well as for externally harvested, domain-relevant data, e.g., from the Deutsche Digitale Bibliothek (DDB). In the future, application profiles will be used for faceted search along with the 2D and 3D previews, to improve the discoverability and accessibility of data for researchers.
In conclusion, the Workbench aims for close integration with the infrastructures of the NFDI4Ing, aligning with the efforts of the FID BAUdigital. This complementarity aims to enhance research data management practices in the fields of civil engineering, architecture, and urban studies and contribute to broader availability and reuse of valuable research data in these domains. Our contribution intends to present the Workbench with its functionalities using representative examples in the context of the FID BAUdigital in order to demonstrate and discuss challenges and opportunities in the work with researchers in the development of application profiles for a domain-specific research data management and infrastructure.
License: CC-BY-SA 4.0 ↗
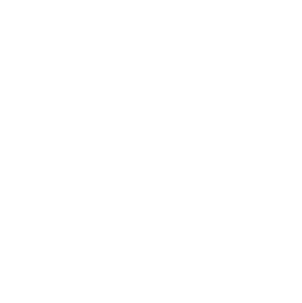
Become a reviewer
Im Rahmen des Community Meeting lobt NFD4Ing einen Award für die beste FDM-Lösung des Jahres 2022 aus. Nominiert werden können alle Lösungen, die nicht im Kontext von NFDI4Ing selbst entstanden sind. Wenn Sie selbst an einer Lösung mitgestaltet haben oder die Lösung andere nominieren möchten, würden wir uns freuen, wenn Sie uns eine entsprechende Nachricht mit dem Betreff “NFDI4Ing Award” an folgenden Adresse senden: community@nfdi4ing.de
- FAIR engineering science: applying principles to practice
- RDM tools and services: usability and automation
- Open science: impact and visibility of engineering insights
- Data literacy in engineering education
visibility of engineering insights
engineering education
Alles auf einen Blick
Everything at a glance
Data is the commodity of the future. In research, FAIR data, data quality and research data management (RDM) are therefore experiencing a steady increase in importance. Likewise, in industry it is important to collect, structure and evaluate data.
Mit der NFDI4Ing Konferenz bringen wir Forschung und Industrie zusammen zum Austausch, gegenseitigen Lernen und Vernetzen.The NFDI4Ing conference brings together research and industry for exchange, mutual learning and networking.
Data is the commodity of the future. In research, FAIR data, data quality and research data management (RDM) are therefore experiencing a steady increase in importance. Likewise, in industry it is important to collect, structure and evaluate data.
Mit den NFDI4Ing Community Meetings bringen wir Forschung und Industrie zusammen zum Austausch, gegenseitigen Lernen und Vernetzen. Um der Vielfältigkeit der Ingenieurwissenschaften gerecht zu werden, fokussieren wir uns hier auf die Community im Maschinenbau und der Produktionstechnik (CC-41).The NFDI4Ing Community Meetings bring together research and industry for exchange, mutual learning and networking. In order to fulfill the diversity of the engineering sciences, this community meeting focuses on the community in mechanical engineering and production technology (CC-41).
Erfahren Sie mehr über aktuelle Entwicklungen des Forschungsdatenmanagements in unseren Themenblöcken "Get a grip on RDM" und "Metadata, Workflows and Ontologies" . Die Veranstaltung ist ebenfalls in diese beiden Slots geteilt, sodass zu jeder Zeit zwei Vorträge stattfinden, jeweils zu einem der beiden Themenblöcke zugehörig. Was Sie in den einzelnen Themenblöcken erwartet können Sie der folgenden Übersicht entnehmen. Darüber hinaus finden Sie ausklappbare Abstracts der Beiträge, indem Sie Beitragstitel mit dem ▶ Symbol anklicken.Learn more about the current activities on research data management in our topic segments "Get a grip on RDM" and "Metadata, Workflows and Ontologies" . The whole event is divided into these two slots, so there will be two talks at any given time, each associated with one of the two topic segments. What you can expect in the individual topic segments can be seen in the following overview. Additionally we have added fold-out abstracts to the contributions. You can find these by klicking the contribution topics with the ▶ symbol.
Wann? | 26. und 27. Oktober 2022, jeweils von 9:00 bis 17:00 Uhr |
Wo? | TBD: Online oder vor Ort an der RWTH Aachen |
Wer? | Alle FDM-Interessierten innerhalb und außerhelb der NFDI4Ing |
Was? | Themen rund um FDM, Ergebnisse und Fragen aus der NFDI4Ing sowie nationale und internationale Gastvorträge |
When? | October 26th and 27th, 2022, between 9AM and 5PM |
Where? | TBD: Online or live at the RWTH Aachen |
Who? | Everyone interested in RDM within and beyond NFDI4Ing |
What? | Topics regarding RDM, results and questions from the NFDI4Ing as well as guest lectures both national and international |
Im Rahmen der Konferenz wurde von NFD4Ing auch ein Award für die beste FDM-Lösung des Jahres 2021 ausgelobt. Nominiert werden konnten alle Lösungen, die nicht im Kontext von NFDI4Ing selbst entstanden sind. Als Teilnehmer der Konferenz bestand die Möglichkeit die Stimme für eine favorisierte Lösung abzugeben. Eine Liste aller nominierten FDM-Lösungen finden Sie hier.
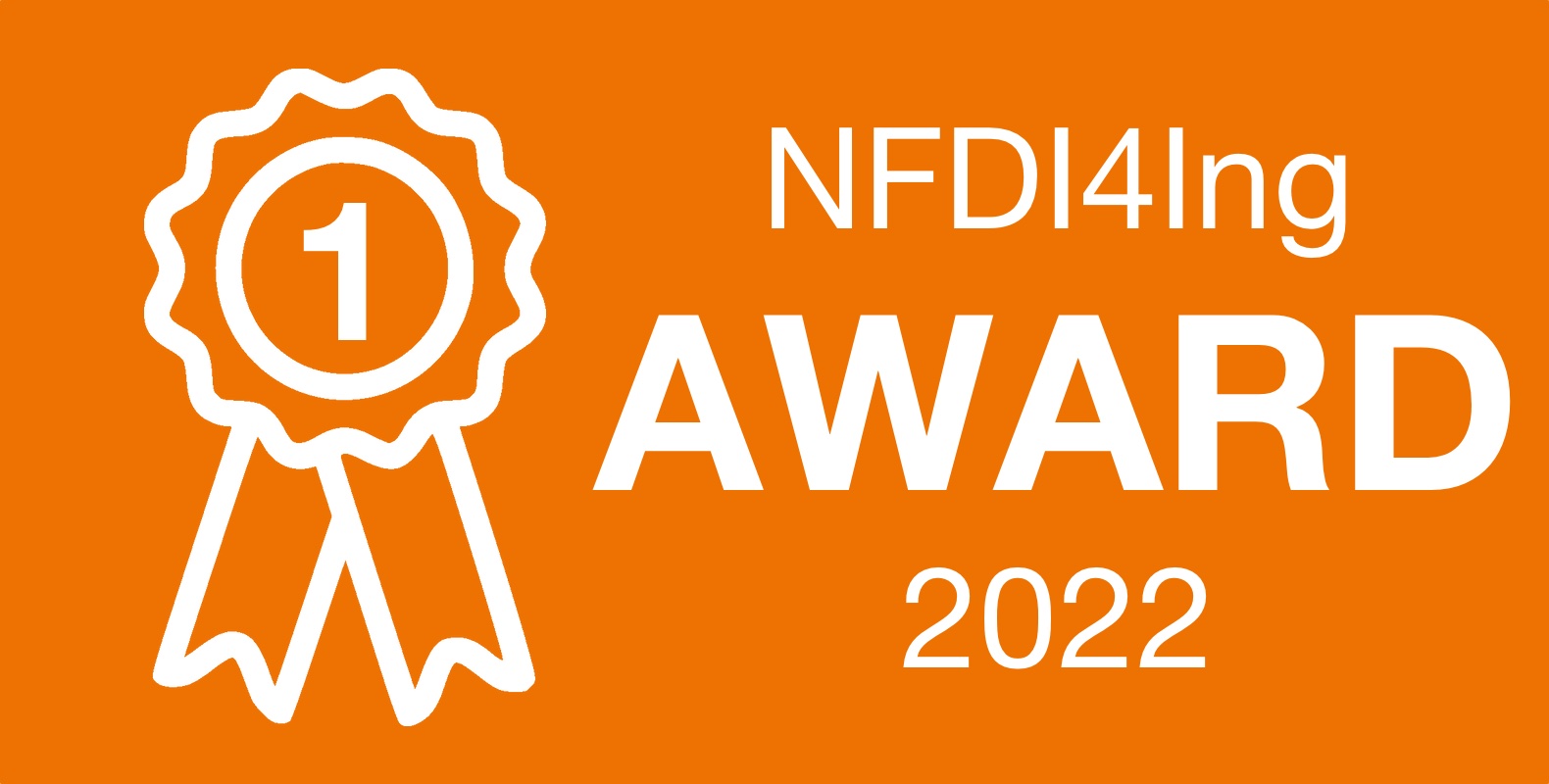
Nominate Your Favorite FDM Solution of the Year!
Im Rahmen des Community Meeting lobt NFD4Ing einen Award für die beste FDM-Lösung des Jahres 2022 aus. Nominiert werden können alle Lösungen, die nicht im Kontext von NFDI4Ing selbst entstanden sind. Wenn Sie selbst an einer Lösung mitgestaltet haben oder die Lösung andere nominieren möchten, würden wir uns freuen, wenn Sie uns eine entsprechende Nachricht mit dem Betreff “NFDI4Ing Award” an folgenden Adresse senden: community@nfdi4ing.de
Im Rahmen der Konferenz lobt NFD4Ing einen Award für die beste FDM-Lösung des Jahres 2022 aus. Nominiert werden können alle Lösungen, die nicht im Kontext von NFDI4Ing selbst entstanden sind. Als Teilnehmer der Konferenz haben Sie die Möglichkeit selbst Ihre Stimme für Ihre favorisierte Lösung abzugeben. Eine Liste aller nominierten FDM-Lösungen finden Sie hier.
As part of the conference, NFD4Ing is announcing an award for the best FDM solution of the year 2022. All solutions that have not been developed in the context of NFDI4Ing itself can be nominated. So if you contributed to a solution yourself or would like to recommend a solution of others, we would be pleased if you would nominate it in the following.
08:45 – 09:00
🎫 Arrival and virtual Check-In
09:00 – 17:00
TBA
08:45 – 09:00
🎫 Arrival and virtual Check-In
09:00 – 17:00
TBA
Get a grip on RDM
Special Interest Groups (SIGs) und Sektionen
Special Interest Groups (SIGs) and sections
Learn more about the cross-topic collaborations within NFDI4Ing as well as the sections that span the entire NFDI. In this slot the topics of RDM Quality Assurance, RDM Training as well as legal and ethics aspects are discussed.
FDM Anwendungsfälle aus den Exzellenzclustern der CC-41
RDM Use Cases in the clusters of excellence of CC-41
FDM in practice will be presented in two exciting use cases: In its workshop, the Internet of Production Excellence Cluster wants to find out what discipline-specific and application-oriented data management plans can look like. Here, the community is called upon to participate! Subsequently, the Cluster of Excellence Fuel Science Center will present the FSC platform, a tool that is used in practice.
Bisher kaum bekannte/ genutzte Tools für die Unterstützung der Forschung
Previously little known/used tools to support research.
Learn in this slot about tools that could help your research. In production engineering, there are also experiments that need to be documented. Electronic lab books are currently hardly used in the CC-41 community, but can be very helpful here. The same is true for the data repository search engine re3data, where CC-41 is hardly represented. We want to change that!
Metadata, Workflows and Ontologies
Special Interest Groups (SIGs) und Sektionen
Special Interest Groups (SIGs) and sections
This slot adresses cross-application collaborations within NFDI4Ing and beyond. Here topics like terminologies, metadata, ontologies, workflows and infrastructures are presented.
Von Terminologien zu Metadaten-Nutzung
From Terminologies to metadata usage
In three consecutive presentations, the Terminology Service will be introduced first, which is used in the AIMS platform. With AIMS on the other hand, application profiles can be created based on existing metadata standards. The data repository Coscine uses those to provide metadata. This results in a toolchain that builds on each other and strives to deliver real value in RDM.
Ontology Prime Time
Ontology Prime Time
Prime Time at the Community Meeting: The "Ontologies Experts Group" of the Cluster of Excellence Internet of Production and fairsharing.org introduce themselves. A must for all ontology fans!
Sonderausgabe | Special issue
Übergreifende Spezialthemen
Overarching special topics
Alle, die sich schon hinreichend über die NFDI4Ing und ihre Aktivitäten informiert haben finden in diesem Beitragstripel Spezialthemen zu dem Journal ing.grid, den FAIR Data Spaces und Hardware.
For those of you who are already well informed about NFDI4Ing and its activities, this trio of articles includes special topics on the ing.grid journal, FAIR Data Spaces, and hardware.
High noon
FDM Anwendungsfälle aus der CC-41
RDM Use Cases in CC-41
FDM zum anfassen gibt es bei diesen drei spannenden Use-Cases!
Get in touch with RDM and our three exiting Use Cases!
High noon
Von Terminologien zu Metadaten-Nutzung
From Terminologies to Metadata-Usage
In drei aufeinanderfolgenden Vorträgen wird zuerst der Terminology Service vorgestellt, welcher später in AIMS eingebunden werden soll. AIMS selbst soll in Coscine anwendung finden. Somit ergibt sich eine Toolchain, die aufeinander Aufbaut und bestrebt ist, einen echten Mehrwert zu liefern.
In three consecutive presentations, the Terminology Service will be introduced first, which will later be integrated into AIMS. AIMS itself will be applied in Coscine. This results in a toolchain that builds on each other and strives to deliver real value in RDM.
Data dawn at dusk
Es gibt kein "Zu viel FDM"
There is no such thing as "too much RDM"
Sie können nicht genug von FDM kriegen? Erfahren Sie mehr über die Möglichkeiten, sich über FDM zu informieren und auszutauschen!
Not enough RDM for your liking? Find out about other offers regarding RDM information and networking!
Data dawn at dusk
Ontology Prime Time
Ontology Prime Time
Prime Time im Community Meeting: Die Ontologies Experts Group des IoP und fairsharing.org stellen sich vor. Ein Muss für alle Ontologie-Fans!
Prime Time at the Community Meeting: The Ontologies Experts Group of the IoP and fairsharing.org introduce themselves. A must for all ontology fans!
Im Rahmen der Konferenz wurde von NFD4Ing auch ein Award für die beste FDM-Lösung des Jahres 2021 ausgelobt. Nominiert werden konnten alle Lösungen, die nicht im Kontext von NFDI4Ing selbst entstanden sind. Als Teilnehmer der Konferenz bestand die Möglichkeit die Stimme für eine favorisierte Lösung abzugeben. Eine Liste aller nominierten FDM-Lösungen finden Sie hier.
Agenda
The CC-41 2022 Community Meeting comes along with the following program of presentations, workshops and networking opportunities:
Thursday, March 3rd, 2022
All times in CET
08:45 – 09:00 Uhr
🎫 Ankommen und virtueller Check-In | Arrival and virtual Check-In
09:00 – 09:15 Uhr
Begrüßung und Vorstellung des Programms | Welcoming and Introduction to the agenda
» Tobias Hamann, Mario Moser (NFDI4Ing Community Cluster CC-41)
(15 Min, )
09:15 – 09:45 Uhr
Neue Werkzeuge für nachhaltige Produktionsforschung
» Prof. Robert Schmitt (Sprecher NFDI4Ing)
(30 Min, )
09:45 – 10:15 Uhr
Vorstellung und Update zur NFDI4Ing | Presentation and Update regarding NFDI4Ing
» Mario Moser (NFDI4Ing Community Cluster CC-41)
(30 Min, )
10:15 – 10:30 Uhr
☕ Pause | Break
10:30 – 12:00 Uhr
Derzeitige Aktivitäten in der NFDI4Ing: Special Interest Groups (SIGs) der NFDI4Ing und Sektionen der NFDI e.V.
Current Activities in the NFDI4Ing: Special Interest Groups (SIGs) of the NFDI4Ing and sections of the NFDI e.V.
Cross-topic collaborations within and beyond NFDI4Ing
10:30 - 11:00 Uhr
SIG FDM Training
» Manuela Richter
(30 Min, )
11:00 - 11:30 Uhr
SIG Quality Assurance & FAIR Metriken
» Iryna Mozgova
(30 Min, )
11:30 - 11:45 Uhr
Sektion EduTrain (Training & Education)
» Manuela Richter
(15 Min, )
11:45 - 12:00 Uhr
Sektion ELSA (Ethical, Legal & Social Aspects)
» Griscka Petri
(15 Min, )
Cross-application collaborations within and beyond NFDI4Ing
10:30 - 11:00 Uhr
SIG Workflow Tools
» Dennis Gläser
(30 Min, )
11:00 - 11:30 Uhr
SIG Metadata & Ontologies
» Susanne Arndt
(30 Min, )
11:30 - 11:45 Uhr
Sektion Common Infrastructures
» Dr. Sonja Schimmler
(15 Min, )
11:45 - 12:00 Uhr
Sektion Meta(daten), Terminologien und Provenienz
» Dr. Rainer Stotzka
(15 Min, )
Cross-topic collaborations within and beyond NFDI4Ing
SIG FDM Training
Research data management (RDM) training and data literacy education is a crosscutting topic within NFDI4Ing and beyond the consortium. While a variety of materials and concepts already exist, it is challenging to select and adapt them to the needs of the engineering community.
The SIG RDM training & education serves to facilitate communication between archetypes, base services and community clusters (CCs) within NFDI4Ing. It also connects to the developments in the NFDI section „Training & Education“ as well as other cross-regional initiatives on RDM training. By creating a channel for communication with the community, the SIG aims to support the measures S-6/CC-2 in their goal of utilization, evaluation and adaptation of materials and concepts for basic RDM training according to the needs of the engineering sciences. Quelle ↗
» Manuela Richter
(30 Min,

SIG Quality Assurance & FAIR Metriken
The SIG quality assurance and metrics for FAIR data provides and discusses standards, metrics and guidelines for organizing data curation based on existing best practices and the FAIR data principles. The SIG focuses on challenges and applications of quality assurance and metrics in the context of NFDI4Ing, but many issues are transferable and apply to many types of data and research data management processes. Furthermore, the methods and tools developed by NFDI4Ing for the self-organization and self-monitoring of quality and maturity of research data and data management processes require an ongoing exchange of experience between different national and international stakeholders. For this reason, close collaboration with researchers in other NFDI consortia and the larger scientific community is a major goal of the SIG. Quelle ↗
» Dr. Iryna Mozgova
(30 Min,

Sektion EduTrain (Training & Education)
Ein besseres Forschungsdatenmanagement im täglichen Betrieb beruht auf den Kenntnissen der einzelnen Forscher:innen. Daher setzt sich NFDI für die Stärkung der Datenkompetenz in universitärer und außeruniversitärer Forschung ein. In der Sektion „Training & Education“ sollen Schulungsmodule mit Lehrmaterialien entwickelt werden, die sich an dem Bedarf der Zielgruppen orientieren. Zudem soll ein Zertifikatskurs für Data Stewards, Personen, die innerhalb einer Institution für eine gute Datenqualität zuständig sind, konzipiert werden. Quelle ↗
» Manuela Richter
(15 Min,

Sektion ELSA (Ethical, Legal & Social Aspects)
Die Bereitstellung und der Austausch von Forschungsdaten sind oftmals mit Rechtsfragen verbunden. Im Umgang mit personenbezogenen Daten muss beispielsweise der Datenschutz besonders berücksichtigt werden, während in anderen Bereichen das Immaterialgüterrecht, also der Schutz des geistigen Eigentums, eine besondere Rolle spielt. Die Sektion „Ethical, Legal & Social Aspects“ soll ein Austauschforum für rechtliche, sozialwissenschaftliche und forschungsethische Erfahrungen bieten. Gemeinsam sollen Leitlinien und rechtliche Standards für das Forschungsdatenmanagement im Wissenschaftsalltag erarbeitet werden. Quelle ↗
» Dr. Dr. Grischka Petri
(15 Min,

Cross-everything: Journals, Data Spaces, Hardware
Journal ing.grid
The journal is committed to the principles of Open Data, Open Access and Open Review. Open Data enhances transparency of scientific processes, accountability of researchers and reusability of research results. Open Access facilitates the dissemination of scientific discoveries, making them operational for addressing societal issues. Open Review nurtures vibrant scholarly discussion.
The journal is firmly rooted in the engineering sciences. It welcomes contributions from all engineering subject areas. Moreover, the journal recognizes connections and common practices across all subdisciplines of the engineering community and encourages active exchange of experiences. Quelle ↗
» Kevin Logan
(30 Min,

Gaia-X meets FDM: Die FAIR Data Spaces
In den Fair Data Spaces werden die föderierte, sichere Dateninfrastruktur Gaia-X und NFDI zu einem gemeinsamen, cloud-basierten Datenraum für Industrie und Forschung unter Einhaltung der FAIR-Prinzipien verbunden. Das heißt, Daten sollen auffindbar, zugreifbar, interoperabel und wiederverwendbar (findable, accessible, interoperable, reusable) geteilt werden. Quelle ↗
» Dr. Marius Politze
(30 Min,

Open.Make - Towards open and FAIR hardware
Abstract: Currently, some grassroots initiatives are pushing to develop an "open hardware strategy for science" that extends open source principles from software to physical products. This is very much in line with the promotion of FAIR data principles. Open.Make aims to explore best practices for creating and publishing research hardware and to develop a prototype publishing platform for research hardware. Lessons learned will also inform open access guidelines to assess and ensure replicability of hardware in science. Given the wider role of academic research in society, a new career path for open and FAIR hardware engineers may also emerge in the future. Quelle ↗
» Robert Mies
(30 Min,

11:30 - 12:00 Uhr
Coming soon
Cross-application collaborations within and beyond NFDI4Ing
SIG Workflow Tools
Software-driven scientific workflows are often characterized by a complex interplay of various pieces of software executed in a particular order. Moreover, each process in a workflow may pose a number of requirements on the software or hardware environment.
In this SIG, we want to elaborate, together with the scientific community, a vision on how scientific workflows should be created, packaged, and published in order to be as FAIR as possible. We want to evaluate if existing workflow tools provide reusable solutions, and identify the capabilities that are missing to reach our goal: reproducible research workflows, by anyone, anywhere and anytime. Quelle ↗
» Dr. Dennis Gläser
(30 Min,

SIG Metadata & Ontologies
The SIG metadata & ontologies supports the exchange between NFDI4Ing Archetypes, Community Clusters and Base Services on cross-sectional topics on metadata and ontologies as well as services using such resources. It allows the different groups to update each other on their progress and requirements and to develop services that meet practical demands in research data management in engineering.
The SIG will develop a metadata model for engineering research metadata according to the community’s needs in its subgroup Metadata4Ing, thereby fostering harmonization activities in NFDI4Ing. It will also follow and contribute to cross-consortia activities related to metadata and ontologies. Its subgroup Metadata4Ing has already hosted a cross-consortia workshop and will continue to collaborate with other NFDI consortia by joining the future NFDI section “(Meta)data – terminologies – provenance” which is currently forming. Quelle ↗
» Susanne Arndt
(30 Min,

Sektion Common Infrastructures
Über eigene, vereinzelte Informationsinfrastrukturen verfügen bereits verschiedene Forschungsdisziplinen. Damit diese heterogenen Angebote in Zukunft interoperabel nutzbar sind und der interdisziplinäre (Daten-)Austausch vorangetrieben wird, sollen diese in eine gemeinsame Infrastruktur eingebettet werden. Angedacht ist die Realisierung einer multi-cloud-basierten Basis-Infrastruktur. In der Sektion „Common Infrastructures“ werden außerdem die Themen nachhaltige Nutzbarkeit und Langzeitarchivierung eine Rolle spielen. Quelle ↗» Dr. Sonja Schimmler
(15 Min,

Sektion Meta(daten), Terminologien und Provenienz
Gemeinsame Standards im Forschungsdatenmanagement sind die Grundlage für die Möglichkeit einer effektiven Nachnutzung von Daten. Die Sektion „(Meta)daten, Terminologien und Provenienz“ arbeitet daran. Entwickelt werden sollen Best Practices zur Modellierung von Terminologien, Vokabularen und Ontologien. Zudem sollen unter anderem einheitliche und nachvollziehbare Dokumentationsverfahren von technischen und kulturellen Aspekten des Entstehungskontextes von (Meta-)Daten etabliert werden. Quelle ↗
» Dr. Rainer Stotzka
(15 Min,

12:00 – 13:00 Uhr
🍝 Mittagspause | Lunch break
13:00 – 14:30 Uhr
Use Cases und Metadaten
Use Cases and Metadata
RDM Use Cases related to mechanical and production engineering on the basis of two clusters of excellence
Workshop: Entwickeln und Implementieren von Datenmanagementplänen für ingenieurswissenschaftliche Projekte des Exzellenzclusters Internet of Production (IoP)
Wie können generische Datenmanagementpläne fachspezifisch angepasst und erweitert werden?
Wie gelingt es, die Datenmanagementpläne innerhalb vorhandener Projektstrukturen zu etablieren?
Diesen beiden Fragen gehen wir mit Input aus Projekten der Research Data Alliance und des Exzellenzclusters Internet of Production auf den Grund.
» Soo-Yon Kim
» Sabine Schönau
(60 Min,

Harnessing the valuable knowledge in the interdisciplinary cluster of excellence "The Fuel Science Center (FSC)"
The FSC-Platform keeps about 30 scientific institutes and 170 researchers up to date on the cluster's research results. Here, the project descriptions and results as well as current publications are displayed in a transparent and standardised form. This enables researchers to see who has published what, with whom on which topic in one or more projects. These information are used by the FSC steering committee to adjust the research direction of the entire cluster with respect to content.
» Robert Jungnickel
(30 Min,

14:00 - 14:30 Uhr
Coming soon
From Terminologies to Metadata-Usage
13:00 - 13:30 Uhr 2P1
Terminology Service
» Dr. Felix Engel
(30 Min, )
AIMS: Applying Interoperable Metadata Standards
Gute wissenschaftliche Praxis erfordert eine präzise und verständliche Dokumentation der Ergebnisse. Forschungsdatenmanagement auf Basis weitreichend standardisierter Metadaten ist daher von essenzieller Bedeutung. Dies ist umso wichtiger, wenn Forschende ihre eigenen Forschungsdaten teilen und publizieren oder archivierte Daten Dritter nachnutzen möchten. Anhand eines Anwendungsfalls aus der Produktionstechnik wird demonstriert wie eine Integration moderner Standards den Forschungsalltag in Zukunft bereichern wird. In AIMS gestaltet ein interdisziplinäres Team die Entwicklung einer Plattform zum Erstellen und Teilen von anwendungsspezifischen Metadatenschemata mit hoher Interoperabilität und Nachnutzbarkeit.
» Nils Preuß
(30 Min,

Coscine – Research (meta)data management made easy
Metadata is a core aspect of good research data management, but data is rarely tagged with metadata in everyday research. The research data management platform Coscine aims to change this by linking the uploading of data to the assignment of metadata.
» Benedikt Heinrichs
» Dr. Ilona Lang
(30 Min,

13:30 - 14:00 Uhr
Coming soon
14:30 – 15:00 Uhr
☕ 💬 Virtuelle Kaffeepause mit Networking-Session | Virtual coffee break and networking session
Grab a coffee and join other interested people in one of our virtual break out rooms.
15:00 – 16:30 Uhr
Vernetzung und Ontologien
Networking and Ontologies
RDM-Tools in Mechanical and production engineering
Elektronische Laborbücher in Physik und Ingenieurwissenschaften
Aktuell schauen viele Institute und Arbeitsgruppen nach Lösungen zu elektronischen Laborbüchern (ELN). Der Markt ist unübersichtlich, und häufig können die Suchenden kaum ihre Anforderungen formulieren. Dieser Betrag zeigt, was ELNs leisten können, welche verschiedenen Ansätze es gibt und wo weitere Hilfe gefunden werden kann. Der Beitrag beschränkt sich dabei auf Lösungen für Physiker, Materialwissenschaftler und Ingenieure.
» Torsten Bronger
(30 Min,

Workshop: re3data – Indexing and discovering research data repositories for the engineering sciences
The service re3data, the Registry of Research Data Repositories, currently lists over 2700 digital repositories across all scientific disciplines and is used by researchers and other services worldwide. A variety of funders, publishers, and scientific organizations refer to re3data within their guidelines and policies, recommending it as a trusted service to researchers looking for appropriate repositories for storage and discovery of research data. Since January 2020, the re3data COREF project has been funded by the DFG to further develop and enhance the registry service.
The talk will give a general introduction as well as a demo of the service, in particular the indexing and curation process. We will take a brief look at the subject classification implemented by re3data and examine which repositories indexed in re3data are associated with institutions participating in NFDI4Ing. We conclude by opening a discussion with the audience with the goal of identifying options to ensure good representation of research data repositories for engineering sciences.
» Robert Ulrich
» Dorothea Strecker
» Rouven Schabinger
(60 Min,

15:30 - 16:30 Uhr (vorläufig)
re3data – Indexing and discovering research data repositories for the engineering sciences
» Robert Ulrich
» Dorothea Strecker
» Rouven Schabinger
(60 Min, tbd)
15:30 - 16:00 Uhr
Coming soon
The Power of Ontologies
Cluster of Excellence Internet of Production: Proposed Ontology for Digital Shadows from Theory to Practice
In this presentation, we present our proposed conceptual model to describe digital shadows that is established via interdisciplinary research in German Cluster of Excellence. Furthermore, we provide a schema and a tool to implement and analyze the proposed framework. This includes data lifting, validation, querying, etc., where the user can validate the schema based on a reference schema and do desired queries.
» Anahita Farhang Ghahfarokhi
(30 Min,

Live-Demo: FAIRsharing: an ecosystem of research standards and databases for effective RDM
FAIRsharing is an informative and educational resource on interlinked standards, databases and policies, three key elements of the FAIR ecosystem. FAIRsharing is adopted by funders, publishers and communities across all research disciplines. It promotes the existence and value of these resources to aid data discovery, interoperability and sharing across all of our stakeholder groups. Here we discuss how FAIRsharing can be searched and updated by our user community, and how you can make the best use out of it as part of a broader data management infrastructure. We will also let you know how you can take part and contribute to the development of the description of engineering resources within FAIRsharing.
» Allyson Lister
(60 Min,

16:30 – 17:00 Uhr
(vorläufig)
Journal ing.grid
» Kevin Logan
(30 Min, )
16:30 – 17:00 Uhr
Ausblick und Verabschiedung | Outlook and farewell
- Feedback
Feedback
- Ausblick auf nächste Schritte
Outlook for next steps
- Zukünftige Angebote zur Vernetzung in der Community und Kontaktmöglichkeiten
Future offerings for networking in the community and Contact
(30 Min,

17:00 Uhr
🏴 Veranstaltungsende | End of the event
ab 17:00 Uhr
🍻 Virtueller Ausklang | Virtual After-Glow
The networking session was too short? Still this one question open? Feel free to stay some minutes longer.
Exited for more? Sign up now!
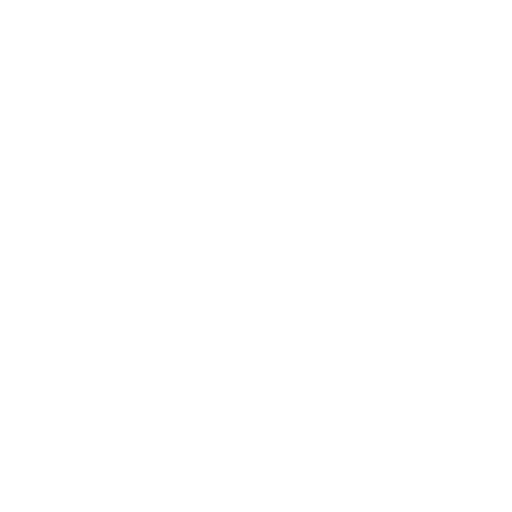
Fragen | Questions
Do you have questions regarding the event? Please use this contact form to get in touch with us!