Field experiments are characterized by environmental conditions that cannot be fully controlled by the experimenter. Examples for field experiments include testing driver assistance systems in traffic, submarine robots in open water, or monitoring traffic in cities.
During data collection, the technical system, its data, and the researcher are influenced by the field conditions such as the weather, the composition of the soil, or the time of day. The documentation of these field conditions, as well as basic information on the experiment, e.g., the people involved in the experiment as well as their role, is elementary for the interpretation of the recorded data and thus for its reuse. Task Area Golo is developing services and solutions to support engineers conducting field experiments in their work.
Data collectors in field experiments have to deal with a great variety of challenges, and a single error might ruin the entire data acquisition. The technical systems that run the experiment tend to have numerous sensors and computers, often producing large amounts of data. The environment may be taxing on the hardware, and critical infrastructure may be scarce. Unpredictable events can influence the data and have to be documented accordingly.
The approaches to dealing with these challenges vary from experiment to experiment. To adequately address the different approaches and requirements for research data management in the field with our services, we conducted a survey in the engineering community. The survey is still running, so feel free to participate (to the survey). Initial responses from more than 60 engineers already paint a clear picture of current research practice: solutions are domain-specific and individual, few standards are commonly known, and there is a great need for schemas and instructions for the documentation of field experiments. In Golo, we are working on multiple solutions to address these findings.
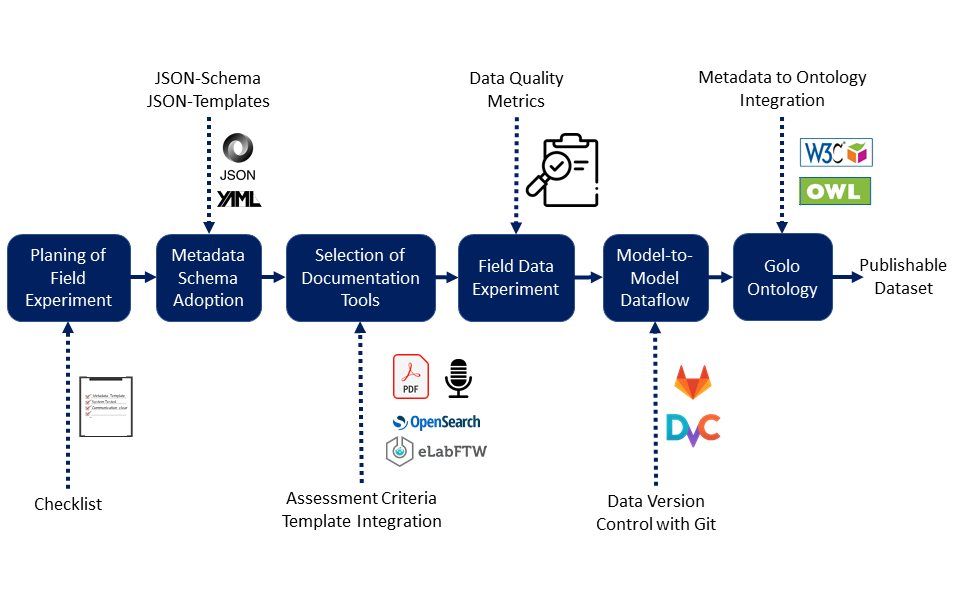
Fig. 1: Workflow for field experiments integrating the Golo results.
Providing a checklist
To support the planning of field experiments, we provide a flexible checklist that can be adjusted to individual requirements and can be developed iteratively over multiple data acquisition campaigns. The checklist addresses the identification of data flows in the field experiment, their documentation, and various data-centric aspects of field experiment preparation.
In addition to the checklist and its implicit methodology, we are creating a metadata schema for field experiments and a guide for collecting the metadata. The guide contains a first selection of tools that can integrate the metadata schema. To support researchers in the selection process, we provide a questionnaire that can be used to extract the key requirements for their documentation tools and then select the appropriate tool. For example, one question addresses the number of documentations required, e. g. when several systems operate simultaneously in a large field. Further possible requirements are, for example, a central, cloud-based documentation tool or independent, individual documentation tools whose documentation can be merged using timestamps after the experiment.
Extending the Metadata4Ing ontology
The services developed in Golo have to meet the needs and requirements of field engineers, while being compatible with other NFDI4Ing services. To achieve the latter, we are in the process of extending the Metadata4Ing ontology. Our extension is designed to divide the research process and its data into individual data-producing steps with implicit temporal relations. Our explicit description of the data sources in technical systems and of the technical systems themselves, based on the SSN/SOSA ontology, enables e. g. searchability for specific data of specific sensors, as well as the integration of machine-readable identifiers of data dictionaries like the IEC 61360. Additionally, information about the field and quality metrics for data, metadata, and datasets can be included. We iteratively compile the quality metrics on a public website, together with application examples for Python, Matlab, and C++.
Interfacing with an ontology is time-consuming and not beginner-friendly. Therefore, we are developing multiple JSON schemas that allow a variety of possibilities for data integration. Data from JSON files can be integrated into the ontology via a Python interface. In the future, support for the YAML file format is planned.
Want to participate?
As we develop our services and test them within our own research projects, we are looking for you as a field researcher to diversify the range of applications. If you want to apply our services in your everyday research and take part in enhancing research data management for field data, feel free to contact us at golo@nfdi4ing.de.
H. Dierend