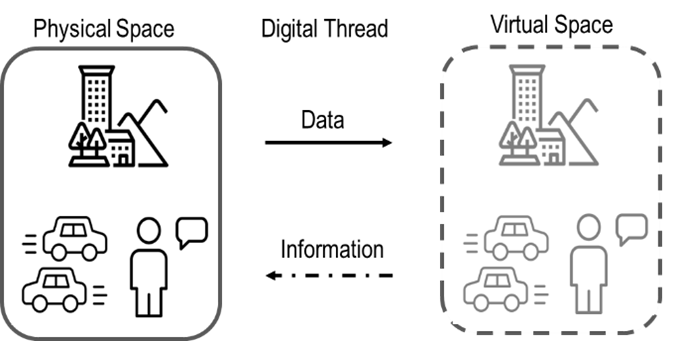
One of the core technologies that we investigate in Golo is the Digital Twin (DT) Framework. Most commonly, a DT framework encompasses three main components that are demonstrated in Fig. 1 [1].
First is the physical space that contains all the physical entities and systems (a.k.a. physical twins) that are of interest. In our investigation in Golo, such systems include complicated machines like vehicles, robots, etc. as well as humans and other aspects of the field environment. This increases the complexity as we need to model interactions among these diverse entities to establish an effective DT framework. The second component of a DT framework is the virtual space that contains a DT, replicating the investigated physical entities. Finally, the third component constitutes the digital thread (a.k.a communication) that connects the two spaces. Significantly, this communication outlines the data flow across the entire framework and facilitates data and information sharing among its different segments.
The primary challenge that we address in Golo is the collection of data from real-world field conditions. Besides, there is uncertainty and a lack of detailed knowledge about the distributed system, and most of the previously established DT frameworks are domain-dependent and cannot be generalized to such field-related cases. Although the concept and specifics of DT frameworks have remained unchanged since their introduction in 2002, there are some prevailing misconceptions among researchers about DTs. The main misconception is that a DT framework is based on an automatic dataflow such that any changes in the physical entity should also change the virtual replica, or that the DT in the virtual space has to be an exact virtual model of a physical entity [2].
In our investigations in Golo, we research a novel DT framework that consists of a digital master that contains all the information for a (physical) instantiation of our physical system, as well as different digital shadows that correspond to the different steps of analysis. Furthermore, we collect data from the field using different sensors and tools that are embedded in a physical instance. The data are later stored, processed, and analyzed in consecutive steps in the proposed DT, directly impacting the potential reusability of the collected information. For more information regarding the ongoing research, please refer to our recent contribution to the NFDI4Ing conference and to our website.
References:
[1] Grieves, M.W., 2023. Digital Twins: Past, Present, and Future. In: The Digital Twin (pp. 97-121). Cham: Springer International Publishing.
[2] Fuller, A., Fan, Z., Day, C. and Barlow, C., 2020. Digital twin: Enabling technologies, challenges, and open research. IEEE access, 8, pp.108952-108971.