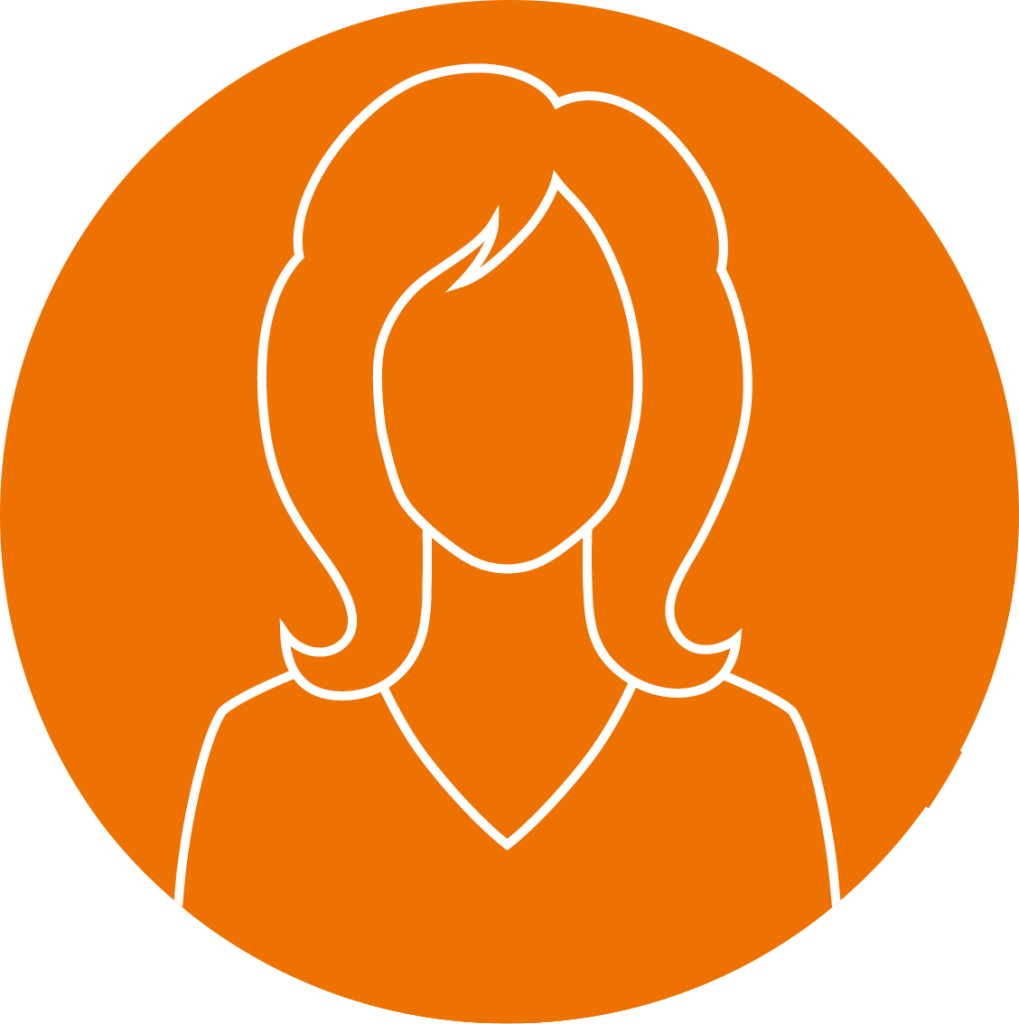
The archetype concept.
Introducing the
archetype:
Ellen
Hello, I’m Ellen.
I’m an engineer who analyses complex systems comprising a large set of multidisciplinary interdependencies. Working within the computational sciences, I do not work in labs, but exclusively on computers and computing clusters. I conduct research by performing model-based simulations and optimisation calculations, whereby I often utilise algorithms coming from statistics and computer science.
Inputs to my analyses are the scenarios I investigate. They typically are very data-intensive requiring information from many different disciplines, such as politics, business economy, jurisdiction, physics, chemistry, demography, geography, meteorology, etc. My professional background is typically based in electrical, chemical or energy systems engineering and is often complemented by several aspects of computer science, physics, and economics.
Key challenges and objectives
A key characteristic of computational sciences are their enormous data requirements. Information from many heterogeneous disciplines has to be compiled. The satisfaction of information needs usually take up a significant amounts of time and has to be repeated in regular intervals. Often, the required information is not available at all in sufficient spatial, temporal or content resolution, has diverging references regarding the object of investigation or is simply outdated. The more of the required data is not available, the more often scientists are forced to resort to inexact estimates and assumptions, which limit the reliability and legitimacy of their research outcomes.
The aim of this task area is to support engineers in their search for data by facilitating established research methodologies as potential data sources, raising their level of integration and reducing the amount of time required for their application. To this end, in the case of unavailable data sets, scientifically recognised methodological concepts and their software implementations will be made available to generate the missing data. Since neither journal articles nor software codes are suitable to be used as a guide to the implementation of a methodology, conceptual and machine-interpretable workflow descriptions will serve this purpose within the research data landscape.
The major objectives of Ellen are to:
1. support scientists’ data retrieval processes by providing the methodological knowledge and the technical means to generate sought data in case it is not found to be available or well suited.
2. develop a semantic framework to enable the representation and reuse of:
a) scientifically recognized methodological knowledge in form of semantically enriched and machine-interpretable concepts.
b) software implementations, software workflows and data sets.
3. enable the engineering community as well as infrastructure service providers to provide and utilize knowledge-based data storage and retrieval services.
Approach & measures
Pursuing the objectives above, the Institute of Energy and Climate Research – Techno-economic Systems Analysis of the Forschungszentrum Jülich (FZJ/IEK-3) and the Leibniz Information Centre for Science and Technology University Library (TIB) lead the activities in the following four measures:
E-1 – Semantic mapping of methodological knowledge: Methodological knowledge will be formalized to semantically rich and machine-interpretable concepts. They will be embeddet into a semantic framework, allowing the retracement and comprehension of complex scientific workflows.
E-2 – Use of methodological knowledge to facilitate data exploration: The methodological concepts will be stored in knowledge graph structures to ensure their findability and accessibility by deploying a concept exploration framework.
E-3 – Connection of data exploration and data generation processes: The automated application of methodological knowledge will be supported by providing web services compiling necessary software and data components. The components will be linked with related information which will jointly be made findable through advanced query algorithms.
E-4 – Community-based validation of data concepts and services: The community will be integrated into the development and validation of the knowledge-based storage and retrieval services. Research findings and best practice guides will be published and disseminated within various community platforms and networks.
Results
Currently the focus in Ellen lies primarily on the creation of a prototype to utilize the ORKG Knowledge Graph structures not only to store and link technology data from energy system analysis, but also to extend them to map information about software models. Publication data has been put into context with energy system model software to pool information on how gaps in datasets can be compensated for by model calculations. First hierarchical template structures have been developed to store metadata from publications, datasets, and energy system analysis software models, thereby serving as the basis for linking information about data generation processes and making it combined discoverable and comparable with each other.
Contact Information
The task area is lead by:
For general information on Ellen please contact: